The researchers at the University of Bonn enhance robotic grasping using neural networks and deep learning. This may soon allow robots to handle objects with human-like finesse, bridging the gap between simulation and reality.
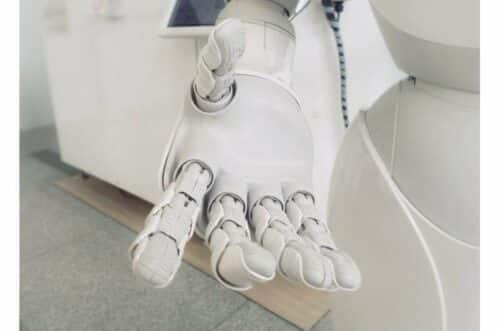
Most adults instinctively know how to grasp and hold objects for their intended use. For example, when grabbing a cooking utensil, they naturally hold the end that doesn’t go into the pot. In contrast, robots must be taught how to grip and handle objects for various tasks properly. This can be challenging, especially when they come across unfamiliar objects.
The Autonomous Intelligent Systems (AIS) research team at the University of Bonn has unveiled a learning framework to enhance a robot arm’s object manipulation skills for functional purposes. Dmytro Pavlichenko, one of the researchers, said, “An object is grasped functionally if it can be used, for example, an index finger on the trigger of a drill; such a specific grasp may not always be reachable, making manipulation necessary. The team crafted an intricate method for dual-arm robotic re-grasping, utilising several intricate hand-designed elements. With this, they address dexterous pre-grasp manipulation with an anthropomorphic hand. The group designed a nuanced strategy for dual-arm robotic re-grasping, employing a series of detailed hand-crafted components.
The team aimed to supplant this intricate pipeline with a neural network, simplifying the process and eliminating hardcoded manipulation tactics, enhancing the method’s adaptability. The streamlined pre-grasp manipulation method utilises deep reinforcement learning, a reputable and effective technique in training artificial intelligence (AI) algorithms. With this approach, the team instructed a model to skillfully handle objects before securing them, guaranteeing the robot grips them as desired. The model learns using a multi-faceted dense reward function, which encourages aligning an object closer to the specified functional grasp through finger-object engagement.
The team posited that their method could be adapted for diverse robotic arms and hands and accommodate the manipulation of various shaped objects. This means it could potentially be trialled on multiple physical robots.
They showcased that emulating intricate human-like actions is feasible with just a single computer and several hours of training. The next research phase aims to transition this learned model into real-world applications, targeting comparable efficiency on an actual robot. Given the complexities, they anticipate that an extra learning phase, conducted directly on the real robot, might be essential to bridge the simulation-to-reality divide.