MIT researchers have used AI to enhance efficiency in robotic warehouses, enabling faster, safer robot coordination and revolutionising complex planning tasks across industries.
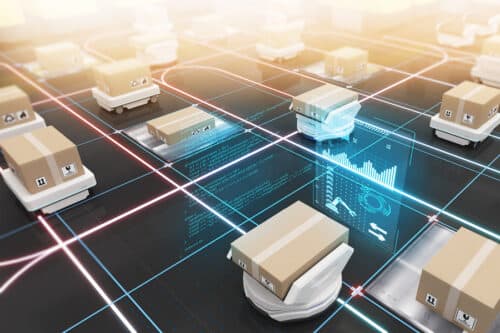
In vast robotic warehouses, hundreds of robots swiftly transport items to human workers for packing and shipping, becoming increasingly common in industries like e-commerce and automotive. However, efficiently coordinating the movement of 800 robots without collisions is a complex challenge, akin to navigating cars through a crowded city centre.
MIT researchers have used AI to improve robotic warehouse efficiency by developing a deep-learning model that predicts the best areas to decongest. They divided robots into smaller groups for quicker decongestion, making their method nearly four times faster than traditional methods. This approach could also be applied to other complex planning tasks, like computer chip design or pipe routing.
Robotic Tetris
In a robotic e-commerce warehouse, hundreds of robots simultaneously retrieve and deliver items, risking collisions if their paths conflict. Traditional algorithms prevent crashes by maintaining one robot’s course while replanning the other’s, but this approach becomes complex with numerous robots and potential collisions.
The researchers have used ML to quickly identify and address congestion areas in robotic warehouses, focusing on regions with the highest potential to reduce total travel time. Their neural network architecture analyses smaller groups of robots, such as 40 out of 800, and predicts which group’s coordination would most improve overall efficiency. The algorithm iteratively selects the most promising group, decongests it with a search-based solver, and repeats the process for the next group.
Considering relationships
The neural network efficiently manages robot groups by understanding their complex interactions, such as potential path crossings. It simplifies computations by encoding constraints only once rather than repeatedly for each subproblem.
In simulated environments, including warehouses and mazes, the researchers’ learning-based approach decongested areas up to four times faster than non-learning-based methods. Even considering the extra computational effort of the neural network, their technique solved the problem 3.5 times faster.
The researchers aim to simplify their neural model into rule-based insights for easier interpretation and implementation in robotic warehouses.