The algorithm, a product of cutting-edge innovation, automates the adjustment of machine feed rates, minimizing the need for manual intervention and significantly cutting production times.Â
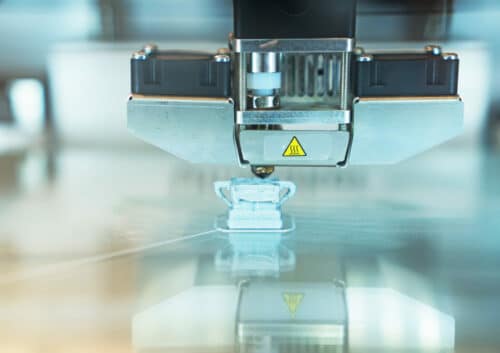
Researchers at the University of Michigan have developed a new method to optimize the speed of manufacturing machines while maintaining quality standards. The study focuses on a digital twin technology that automates the optimisation of machine feed rates.
The researchers drew inspiration for this work from their experience in the manufacturing automation industry. They observed that manufacturers often relied on trial and error to fine-tune machine settings, which was time-consuming, or they chose a conservative approach that compromised productivity to ensure quality. The new algorithm aims to eliminate the need for manual adjustments by allowing machines to automatically find the most productive settings within set quality constraints. This approach has demonstrated significant improvements in production efficiency in tests conducted with a 3-axis desktop CNC machine tool and a desktop 3D printer. The cycle time required to produce a unit was reduced by 38% and 17% respectively.
The method employs a digital twin, which is a virtual model that replicates the real system’s behavior. This model incorporates physics-based principles and real-time data from sensors to predict and compensate for variables that physical models alone might not account for, such as environmental variations. A significant advancement of the algorithm is its ability to manage uncertainty. The digital twin can estimate the probability of producing parts that do not meet quality standards and adjust the machine’s speed accordingly. This feature allows manufacturers to choose their level of risk tolerance; for example, a more aggressive setting might permit a higher percentage of parts to fall below quality thresholds to maximize speed, while a conservative setting would minimize such risk.
Heejin Kim, a doctoral graduate of mechanical engineering at the University of Michigan and the first author of the study, along with Rael Al Kontar, also from the University of Michigan, contributed to the research. The team plans to further enhance the model by integrating more complex uncertainty distributions, aiming to broaden the applicability of their algorithm to other manufacturing scenarios. This advancement represents a promising step forward in the integration of machine learning and real-time data analytics in manufacturing.