Their focus is on facilitating creation of wearables that can record electrocardiograms to prevent stroke due to irregular heart rate
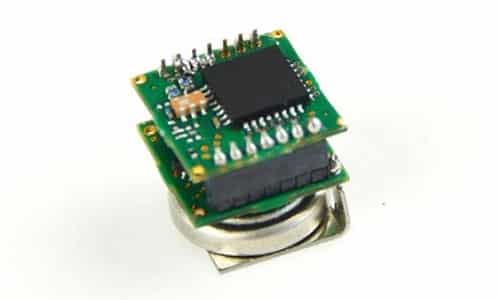
Atrial fibrillation is one of the most common types of cardiac arrhythmia. If not detected in time, the condition can trigger a stroke. Wearables that can record electrocardiograms (ECGs) over a long period of time are a good way to increase the detection of irregular heart rhythms. But there must be a possibility to analyse the recorded ECG data in an energy-efficient manner for mobile diagnosis to be practical. Algorithms for evaluating patient data can be quite computationally intensive, resulting in high energy consumption. Therefore the highest priority for mobile applications should be energy-efficient while evaluating such algorithms.
To promote a viable solution for the above problem, an innovation contest titled “Energy-efficient AI systems” was organised by the German Federal Ministry of Education and Research (BMBF), wherein the participants had to design an AI chip that detected atrial fibrillation with at least 90 per cent accuracy and consumed little energy. Amongst the huge number of participants, Fraunhofer IIS and Fraunhofer ITWM were declared winners, although in different categories and with different approaches.
Signal processing falls into sleep mode
To determine whether the patient is healthy or sick, the Fraunhofer IIS researchers developed the project “Low-Power Low-Memory Low-Cost ECG signal analysis using ML algorithms (Lo3-ML)” that relied on deep learning. A digital ECG signal was used as input into the neural network, the signal sections were filtered, the individual signal components were weighted (with ternary weight values +1, 0 and -1) and summed up in several layers. “In the first layer of the neural network, a certain signal behaviour was detected. In the second layer, the characteristics were placed in relation to each other. A total of six layers were used. A complex image of the ECG signal indicated an existing disease, which did not emerge until the last, sixth layer,” said Marco Breiling, a scientist at Fraunhofer IIS.
To process these time-series signals for energy efficiency, i.e. the digital representation of the ECG signal, the signal processing was made a part of the AI chip to sleep when not needed, thus saving 95 per cent of the energy. “The chip collected the ECG signal for 12.7 seconds and then processed it in just 24 milliseconds, or 0.2 per cent of the time. So the processing sleeps for over 99.8 per cent of the time and uses an almost negligible amount of energy. Thanks to non-volatile RRAM memories that are part of the system, signal processing can resume immediately after wake-up, after almost 12.7 seconds without consuming any energy,” explained Breiling. He further stated, “The chip requires little power such that a solar cell with an area of 6 mm x 6 mm operating in the moonlight would suffice. Alternatively, the chip could evaluate ECGs for 330 consecutive days using a very small coin cell.” The developed circuit is not only suitable for medical use, but also for other applications where time-series signals are processed, such as condition monitoring and predictive maintenance.
Holistic AutoML for FPGAs
The research team at Fraunhofer ITWM took into account both the hardware energy consumption and the neural network topology that offered not just classification accuracy but was also energy efficient.
But how exactly can the networks meet the defined requirements and specifications? “There are different search strategies in this regard. We used an evolutionary approach in which we selected ten different random networks, trained them and checked how well they worked. We then selected the best networks and mutated them to create new network variants. The process was repeated until the best network was found. This procedure is called automated machine learning,” explains Dr Jens Krüger, who conducts research at the Competence Center — High Performance Computing at Fraunhofer ITWM.
Krüger and his team used Field Programmable Gate Arrays (FPGAs) for mapping the neural networks, implementing a variety of circuits and achieving the best possible execution of an optimal algorithm. The FPGA can be reprogrammed any number of times and distinguished by various characteristics that define an optimal neural network. Using a software tool, the neural network was transferred to the FPGA, which then automatically evaluated the ECG data. This approach resulted in a new methodology that was not only more energy-efficient but also reduced the development time for optimal neural network topologies and corresponding FPGA implementations. The software tools developed are suitable not only for FPGAs but also for a wide variety of chips and environments.
The researchers are extending this process to include a holistic approach that considers not only the neural network but also the hardware since the AI model influences the energy consumption of the hardware.
In conclusion, it can be said that Artificial intelligence (AI) can only create benefits and find its way into medical, industrial and other applications if the energy consumption of today’s microelectronics is reduced.