Researchers have designed a 3D-printed robotic hand capable of performing complex actions such as gripping objects and preventing drops through wrist motion.
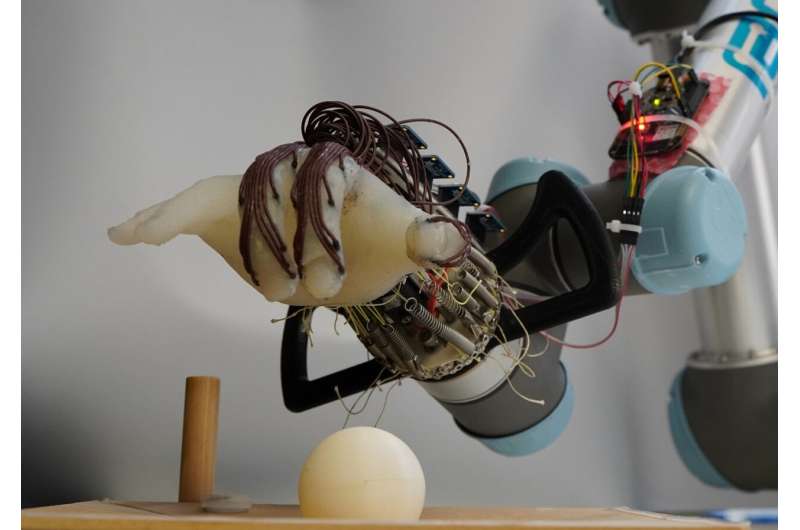
The ability to grasp objects of varying sizes, shapes, and textures is a fundamental skill that humans acquire early in life. However, replicating this skill in robots has proven to be a significant challenge.
Researchers from the University of Cambridge have designed a low-cost, energy-efficient, 3D-printed soft robotic hand that can grasp objects and avoid dropping using wrist movement and “skin” sensation. The trained robot’s hand uses sensors placed on its “skin” to predict the dropping of grasped objects. Recreating human hand dexterity in robots is challenging due to the complexity, manipulation skill gap, and high energy demand of actuated hands.
Robots face difficulty picking up objects, as they require delicate force control. Fully actuated robot hands demand substantial energy and have motors for each joint in every finger. Hence, researchers have created solutions for two problems: a robot hand that can grip objects with proper pressure and minimal energy. They utilized a 3D-printed hand with tactile sensors for sensing, limited to passive wrist movement. The team conducted over 1,200 tests to check the robot hand’s ability to grip small objects without dropping.
Initially, it was trained using predefined actions demonstrated by humans with 3D-printed plastic balls. The developed hand has some springiness and can lift objects without finger actuation. Tactile sensors enable the robot to sense its grip’s efficacy and predict potential failures.
The robot used trial and error to learn successful grips, then tested with various objects, grasping 11 out of 14 items. Sensors are akin to robot’s skin, gauging pressure on the object. The robot can estimate the grasp’s location and force intensity. Robot learns from failed attempts, resulting in a customizable solution. The simple hand picks up many items with a single strategy. Design’s significant advantage is its actuator-free range of motion. Simplifying the hand is our goal, with information and control achieved without actuators.
Adding actuators would provide greater complexity in an efficient package. A fully actuated robotic hand needs more energy and complex control, unlike the passive Cambridge-designed hand, which utilizes few sensors, facilitates control, offers ample motion, and simplifies learning.
Researchers believe that the system may expand in the future by incorporating computer vision or training the robot to utilize its surroundings, enabling it to grip a broader array of objects.
Reference : Predictive Learning of Error Recovery with a Sensorised Passivity-based Soft Anthropomorphic Hand, Advanced Intelligent Systems (2023). DOI: 10.1002/aisy.202200390