Emerging technologies like artificial intelligence (AI) are growing worldwide at an unprecedented pace. Johanna Pingel, marketing manager, deep learning, MathWorks, discusses the good practices that students, researchers, and engineers can follow to accurately solve complex problems using these revolutionary technologies in an interaction with Ayushee Sharma.
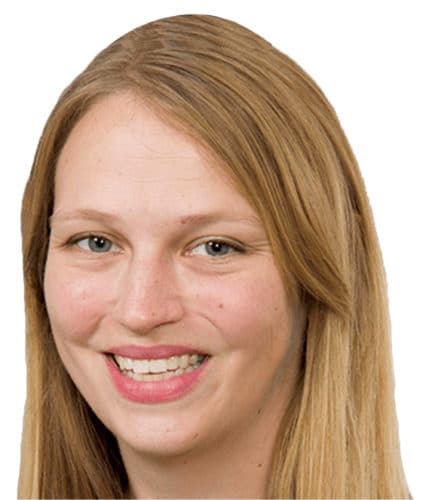
Q. Choosing the right tools/environment to easily work with teams is very important. But with so many choices in the market, what are some of the factors a team should consider?
A. Discussions around AI tools and technologies should include the role of modelling and simulation in the overall workflow. As AI is trained to work with more sensor types (IMUs, LiDAR, radar, etc), engineers are driving AI into a wide range of systems, including autonomous vehicles, aircraft engines, industrial plants, and wind turbines. These are complex, multi-domain systems where the behaviour of the AI model has a substantial impact on the overall system performance.
In this world, developing an AI model is not the finish line; it is merely a step along the way. Today, designers are looking for tools that support Model-Based Design for simulation, integration, and continuous testing of these AI-driven systems. The Model-Based Design represents an end-to-end workflow that tames the complexity of designing AI-driven systems. Simulation enables designers to understand how the AI interacts with the rest of the system. The integration allows designers to try design ideas within a complete system context. Continuous testing allows designers to quickly find weaknesses in the AI training datasets or design flaws in other components.
Q. Reliability is a big factor when it comes to end-user adoption. What are the measures that data scientists and other developers should take to ensure reliability?
A. According to several analyst surveys, data quality is a top barrier to the successful adoption of AI. We know training and testing accurate AI models require lots of data to ensure a reliable system. While there are often lots of data for normal system operation, what’s really needed is data from anomalies or critical failure conditions to ensure the system behaves as you expect in all conditions. This is especially true for predictive maintenance applications, such as accurately predicting remaining useful life for a pump on an industrial site. Since creating failure data from physical equipment would be destructive and expensive, the best approach is to generate data from simulations representing failure behaviour, and use the synthesised data to ensure an accurate AI model.
Q. How is MATLAB used for AI?
A. MATLAB supports the complete AI workflow, from data preprocessing to deployment. Teams with limited machine learning and deep learning experience can still be successful with AI in MATLAB by using apps to quickly try out different approaches and apply their domain expertise to clean and preprocess the data and create AI models. Teams can also take advantage of pre-trained AI models and functionality built by AI experts to quickly build a prototype for their specific application with the data they have. Finally, they can deploy the model as part of a complete AI system on an embedded device.
Q. How do testing and measurement toolboxes ensure/incorporate interoperability among different vendors?
A. There are many tools out there for AI-related applications, and one primary concern of engineers and scientists is vendor lock-in when developing applications. Interoperability ensures teams can design and develop AI algorithms in multiple platforms and still work cross-functionally as a team. With MATLAB, teams working on AI projects can import AI models from many open-source platforms, continue developing algorithms with AI apps and visualisation, and then deploy imported AI models to embedded or GPU devices.
Q. There has been a huge growth in people wanting to pursue roles in emerging technologies. But how can the skill gap be reduced?
A. As AI becomes more prevalent in the industry, more engineers and scientists—not just data scientists—will work on AI projects. They now have access to existing deep learning models and accessible research from the community, which allows a significant advantage than starting from scratch. While AI models were once mostly image-based, most of these are now also incorporating more sensor data, including time-series data, text, and radar.
Engineers and scientists will greatly influence the success of a project because of their inherent knowledge of the data, which is an advantage over data scientists who are not as familiar with the domain area. With tools such as automated labelling, they can use their domain knowledge to rapidly curate large, high-quality datasets, which increase the likelihood of an accurate AI model, and therefore a higher likelihood for success.