When we look at an object, our brain immediately knows how to pick it up – whether to use our palm, or just fingers, or maybe both hands! This sort of intelligence is what is lacking in current robotic systems. CynLr, a Bengaluru-based robotics deep-tech startup founded in 2019, is now solving this problem using a unique approach. In an interview with EFY, Gokul NA and Nikhil Ramaswamy, co-founders of CynLr, talk about how they are working towards achieving visual object intelligence for industrial robots.
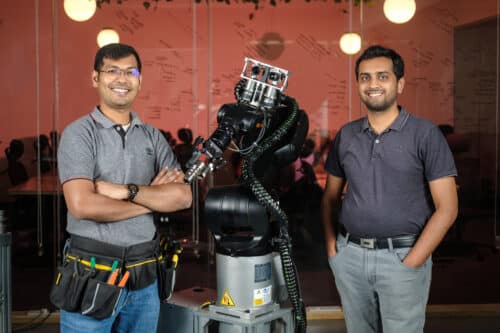
Q. CynLr stands for Cybernetics Laboratory. Could you elaborate on why it was named this?
Gokul NA: Cybernetics is a counter-thesis to typical AI and black-box ML approaches. AI is a very collective term, and there are different structures to it. We usually treat AI like it is a black box – we think that there is some monolithic block of intelligence – like our brain, which we assume is made of very similar neurons put together in a sophisticated way. If you feed inputs to this “brain”, we assume that it automatically trains to create a “template of the input” in order to give the output. When we go behind this template training, we do so with an assumption that intelligent systems or AI “know” their inputs. We assume that they are self-aware systems.
Well, there is no such “one monolithic system.” Cybernetics, in fact, precedes AI as a structure. We often confuse intelligence with the ability to perform phenomenal tasks or handle sophisticated things. However, according to the field of cybernetics, intelligence is the outcome and not the source or cause.
“Intelligence is the ability of a system to self-regulate and handle a sophisticated situation.”
Remember those enormously large and picturesque flocks of birds moving in unison like one intelligent organism, forming extremely complex patterns? Or the large schools of fish that swiftly turn together in a complex way to confuse the predators, like a single intelligent being. Not every fish or bird saw the predator. They just loosely followed the one ahead of them, and yet they ended up achieving very complex behavior.
Ants, locusts, and many other living beings exhibit this collective behavior, which is “not just swarm intelligence” but more fundamental to even “seemingly non-swarm organisms” like the human body. A cell in the liver doesn’t realize that it is reacting to a command from a neuron in the brain, and neither does the neuron in the brain realize that it’s sending a command to the liver. Even a neuron doesn’t realize that it’s reacting to another neuron. And here we are – two bundles of these simple cells curiously discussing all this!
Behind every such complex & intelligent behavior, there are simple systems just ‘adamantly’ trying to achieve ‘a primitive’ fixed task. Interestingly, when you put those simple systems with each other, that adamancy forces them to rearrange and constantly adjust to each other’s stimuli. The result – they end up solving a complex situation, without any awareness of the bigger picture, creating an appearance/illusion of intelligence.
If you look at your brain, the part that handles sight keeps doing image processing, whereas the audio portion does certain kinds of audio processing. When they come in tandem with each other, there’s a sophisticated handling of our reaction to sound and sight. This convergence of these two faculties gives meaning to each of them. The moment I say orange, you are able to visualize the picture of an Orange (the fruit) or the color orange, and this gives meaning to the sound of the word orange – that’s vision. This approach is called cybernetics – the ability of a system to be self-regulatory.
“This ‘self-regulatory’ behavior is cybernetics.”
Nikhil Ramaswamy: The second part of our name comes from labor. Most people don’t know this. A laboratory literally means a place where labor happens. The meaning has changed over time. But basically, robots are an alternative to human labor. And that’s why the name is Cybernetics Laboratory.
Q. You develop industrial robots, or to be precise, visual object intelligence for industrial robots. What exactly is meant by the term “visual object intelligence”?
Gokul NA: Unlike every other technology, vision has kind of become over-assumed and vocabulary-insufficient. When we have to make a system go and interact with an object, like when a robotic arm in an industrial setting needs to pick up an object, it first needs to be able to recognize the object visually. But we tend to limit vision to color and depth. An object that has so many features like weight, shape, and texture gets reduced and abstracted down to only a color pattern. But that’s not enough for the robots to successfully manipulate objects. That is the limitation that we are trying to solve.
Another common misconception is the confusion between sight and vision. Let’s say I give you an object in your hand. You have never seen this object before. You don’t know what it is, you’re not able to identify what it is. So you pick up that object and start tilting it, rotating it, and manipulating it. So you have manipulated that object even before you know what this object is. This is where we confuse sight with vision. Sight gives you a certain amount of data on a 3D object, but vision gives meaning to that data.
“Vision is beyond color and depth.”
Like I mentioned before, when I say the word orange, you start imagining all the other feelings with your associated senses – the texture, smell, taste (you might start salivating). All these associated feelings give meaning to the object. All that comes together only with imagination, the holistic understanding of that object being associated with every other sensory feeling that’s needed for you to be able to interact with it. That is intelligence, and that’s when vision actually happens. That’s what we mean by visual object intelligence. This intelligence, when in robots, allows them to grasp any object without any prior training. Because of this, robots can learn to operate items in any orientation, in addition to grasping objects in unstructured environments.
Q. How did you come up with the idea that’s behind CynLr? What was the first incident that prompted this whole discussion?
Nikhil Ramaswamy: Gokul and I have known each other for about a decade now. We’re both electronics and instrumentation engineers and were both working in the same team at National Instruments (NI). NI is a tech giant headquartered in Austin, Texas. The company makes test and measurement equipment, as well as automation hardware and software. One of NI’s product lines is machine vision, for which they made smart cameras and software libraries that customers, especially from industrial scenarios (like manufacturing plants), could use to put together a variety of machine vision applications.
Machine vision, at that point in time, and even now, was largely synonymous to inspection. People used cameras to identify a scene or an object in the scene and then make some decisions based on that, mostly for quality control purposes. For example, scanning a barcode is a machine vision application. There were many companies that focused on machine vision, including NI and Cognex. Later, as I took a sales role at NI, I got great exposure to what it takes to build large manufacturing plants and large industrial setups. Gokul specialized in machine vision. On the technical side, he ended up becoming the only machine vision specialist for a fairly large geographic area for NI.
We realized that the existing machine vision systems were only successful for simple identification problems. Machine vision was actually notoriously very difficult to solve – if customers had 10 requirements, the existing systems at that time would succeed in only 3 out of the 10 use cases. These systems were not meant for industrial robotics applications. They could not be used by robots to correctly grasp objects, pick, re-orient and manipulate them, and make proper placements in an industrial environment. Imagine a pick-and-place robot that’s not able to grasp an object it’s supposed to pick!
Machine vision for object manipulation had to be treated differently. The paradigms, algorithms, and architectures that were developed in machine vision, generally, were all for identification. People were trying to force-feed those algorithms for manipulation applications, and that was not working. That’s when we started figuring out how we were going to solve this problem of vision for object manipulation. In 2015, we left our jobs and approached customers we knew had a problem to be solved. Over the next few years, we ended up solving over 30 unsolved machine vision solutions that involved some kind of manipulation!
Over time, we have achieved a certain level of standardization. We raised a seed round of funding, and with that funding, we built the hardware infrastructure. We also worked on the customer pipeline and built a team. And earlier this year, we closed our Pre-Series A to commercialize and take this technology to market. So this has been our journey.
Q. Could you elaborate on the hardware and software aspects of your company?
Nikhil Ramaswamy: One of the taglines that we write on our walls is, “Hardware is a part of the algorithm.” On the vision side, especially, we have built our own hardware. In fact, at a hardware level, we are the only depth perception system in the world to have eye movements, convergence, divergence, precision control, autofocus, etc that’s enabled through hardware.
Gokul NA: Software cannot do magic. It’s physical elements and physical objects that we’re actually dealing with. So, without structuring the system to be able to pick or capture information about an object, we cannot create a holistic model of the object or intelligence about the object. Also, information about an object cannot be gotten just from a camera. For example, we think autofocus just brings objects into focus, but actually, it is the first layer of depth construction that we do. There are a lot of missing features in the way we use these physical devices like cameras. So, our inference algorithm begins with the hardware itself, it doesn’t start with the software layers.
Q. What would you say is the most unique feature of your technology?
Gokul NA: Today, human labor is a straightforward process, because the same person with the same infrastructure, or without any infrastructure and additional investment, can go and work in a garment factory, and stitch clothes. The next day, he can work at a different factory to put parts together to make an engine. On the third day, he can go into construction and start putting bricks together. He uses the same hands, eyes, and brain to handle all of these objects.
In the industry today, if you want to flip an object from one position to another, (like from vertical to horizontal), you need to have an insane amount of infrastructure – around 30 to 40 different technologies are needed. That amounts to a lot of money. It also doesn’t mean that the next day, I can reuse all of that infrastructure. What if I want to flip the object in a different orientation? This will be an entirely new set of 30 different technologies! However, the customers who utilize our tech don’t have to change the technology structure or the product structure.
Q. What kind of electronics is used in your products?
Gokul NA: Just like an iMac has a processor from Intel and a screen from some other vendor, only a portion of our systems are made entirely in-house. For most commercial products, it’s the same case. Since we are at a seed stage, our core tech – vision – is the hardware stack that is completely built in-house. For the customers, we bring in a lot of other technologies – the robotic arm, the gripper, some software layers, the processing elements that are to be custom designed for the industrial scenario, etc. We provide our customers with the whole system – a robotic arm equipped with vision and with sophisticated grasping capabilities so that it can handle different kinds of objects.
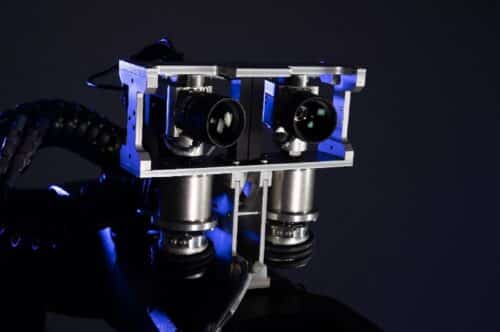
Nikhil Ramaswamy: It’s a sophisticated, human-like camera setup. Many of our parts are custom-designed for us. Our components come from all over the world. So typically, the first version is an amalgamation of technology that originates across the world and comes into that one small focal point of the camera that we design. Eventually, we’ll have manufacturing operations to streamline the production.
We’ve already launched our next iteration, where we will be making it a lot more compact. We’ll keep having newer versions of the camera where we either improve the specs, reduce the size and dimensions, or build versions that can allow us to scale to newer application use cases.
Q. What has been your commercialization story?
Nikhil Ramaswamy: We are actually commercializing as we speak. The good thing is that for what we’re building, the customer and market requirements are clearly established. A robot that has the intelligence to pick an object and do something with it has been a dream for almost 40 or 50 years, ever since the first robotic arm was built.
From the customer’s point of view, and a commercial standpoint, we’ve usually found very little resistance. The challenge is on the supply side of it because there are a lot of parts that come from all over the world. So that’s something that we are actively working to iron out in this space. We are setting up a sophisticated supply chain process, and we already have an ERP system in place. We’re also investing in SAP-like tools so that we can manage all the supply chain issues in manufacturing and delivery. We already have a pipeline of customers and an OEM partner. The road to commercialization is underway.
Q. What kind of challenges have you faced throughout your journey so far?
Gokul NA: On the technology side, the lack of awareness and narrative are challenging. On any media front, the term “machine vision” is uncommon. For example, on LinkedIn, #machinevision will not have as many followers as #computervision. So that is the perspective awareness variation, and this influences the supply chain too. Also, the amount of time that you spend explaining that foundational concept increases, right?
People often get confused between technology and application. Applications are easier to articulate because you’re building on top of a technology that is well established. You don’t have to explain what the Internet is. Because people already know what the Internet is! You don’t have to explain what an Android phone is because people already have an Android phone in their hands. But imagine a company like Xilinx or Nvidia trying to explain what a GPU is in the 1980s and 1990s. It would be difficult. So, a foundational technology company like us always faces this issue. That’s why we are a long-term game.
“Fortunately, for us, customers are well aware of the problem. There are so many places where the tech can be applied, and we are getting interest from different quarters.”
Q. What kind of partnership opportunities are there for like-minded firms to partner with CynLr?
Nikhil Ramaswamy: We are absolutely open to partnerships. We, being a technology company, are going to depend on a fairly broad network of partners, who would either be custom system engineering companies, or productized companies like Ace Micromatic Group. Also, the last mile delivery is going to be done by system integrators. Overall, there are two types of partnerships here – technology partnerships and application partnerships.
Gokul NA: Any technology can be brought into usefulness only through an application. So we are open to all application partnerships. Our tech can be applied to part tending, part assembly, and part inspection from an application perspective. On the other hand, there are technology partnerships that help us enhance our features. For instance, we could partner with vision manufacturers, camera manufacturers, robotic arm manufacturers, or even augmentation systems.
Q. What are your general hiring trends?
Gokul NA: We have grown from an eight-member team to a twenty-member team in this last quarter. By the end of this year, we will have a fifty-member team. So we’re actively recruiting for a wide variety of roles. There is a fair split between the engineering roles and the non-engineering roles. As we speak we are gearing to visit top engineering colleges in the country to recruit for fresher roles.
We hire freshers as well as experienced professionals. Simply put, there are two kinds of problems – unexplored problems and unsolved problems. Unexplored problems are easier to solve, for which you can actually get industry best practice experts. Unsolved problems are where people have a lot of opinions. So it’s harder to make them unlearn and then relearn, which is why we hire people who will have a fresh perspective about things. When it comes to certain platforms, tools and technologies, we try to bring in experts.
“If you see the books that are there in our library, you will see a wide gamut of books, even medical books or legal books!”
Nikhil Ramaswamy: On the engineering side, being in the robotics and vision space, there’s a very broad spectrum of roles. We have all the disciplines coming together. So we hire electronics engineers, mechanical engineers, software engineers, instrumentation engineers, and people with a science background. Some of our engineers are currently working on neuroscience and neuroscience research, trying to validate our algorithms with how human vision works.
Q. What’s next for CynLr?
Nikhil Ramaswamy: As a part of this commercialization plan, we have a goal to establish our presence in at least one country along with India. We’ll be looking to expand to the US, Germany, and western Europe in the future. In India, we are expanding our headquarters in Bangalore and moving to a much larger space. We also aim to have an Experience Center for customers to come and experience what the future of manufacturing is going to look like.
Gokul NA: We’re also looking for domain expansion. Right now, predominantly, our customers are from the automotive sector. In the future, we also want to expand into the electronic assembly market, especially mobile and gadget manufacturing, and even logistics.