Artificial Intelligence is becoming quite popular nowadays as it gives us significant technological power. However, it is important to use it with more responsibility to overcome its disruptive impacts such as loss of privacy, and increased biases in decision-making. Responsible AI would ensure ethical and transparent use of AI technologies.
In my view, tools and systems are not hurtful; people using them are! The thing with technology is if you learn to use it, it works for you. But, ‘who’ you are decides ‘how’ you use it.
One of the things that distinguish humans from others is our freedom to decide how we act. Whatever we choose, we could always have chosen differently.
Responsible artificial intelligence (AI) is not a better technology—it is how we choose to make it and use it.
AI is giving us significant technological power, which begets greater responsibility.
These days, AI is raising more concerns because of the potentially disruptive impact it can create on many fronts. Matters such as massive workforce displacement, loss of privacy, increased biases in decision-making, and general lack of control over automated systems or robots. These issues are significant, and they are also addressable—if we approach with the right planning, oversight, and governance.
Responsible AI is a framework for bringing several critical practices together, where we can ensure ethical, transparent, and accountable use of AI technologies. Their use in a manner that is consistent with user expectations, organisational values, as well as societal laws and norms.
For example, under normal circumstances, mis-selling, especially in the financial services domain, is dealt with utmost caution. The government would step in if the consequences are grave. If a person, i.e., a human does it, you may reprimand them or have some consequences attached. How would you do that when so-called intelligent chatbots are being used to sell something to customers? Suing the salesperson is already stressful, can you think of doing that to a chatbot? Mis-representation is about what a person said to you. What happens when we’re not being sold to by a human at all?
So, understanding and acknowledging that these types of scenarios may exist with your product or service and taking adequate steps to deal with them in the ethically right manner is Responsible AI.
The foundational elements of Responsible AI
Some of the foundational elements have to be timeless and deeply rooted in organisations’ fabric.
Elements such as—moral purpose and society benefit, accountability, transparency and explainability, fairness and non-discrimination, safety and reliability, fair competition, and privacy are part of foundational elements of Responsible AI.
Some of the elements also come from the supply side of AI systems. We see AI vendors continually pushing for faster adoption of AI solutions. I think this is greedy and not at all customer-centric approach. I can understand that vendors want to sell AI by making it easier and frictionless, but they seem to ignore inherent risks in their system as well as their solutions’ ecosystem that is not in vendor’s control. It is becoming more like a cart before the horse situation.
It only benefits vendors, while enterprises often lose money and time. Because once companies start, there is no turning back. So they go deep in the rabbit-hole and keep bleeding through the nose. Cautious steps are of utmost necessity, by vendors as well as by enterprises.
However, in general, I think, it must cover three core aspects of a good solution design, i.e., you are designing the right solution, the solution has been designed right, and most importantly, you have covered all the risks.
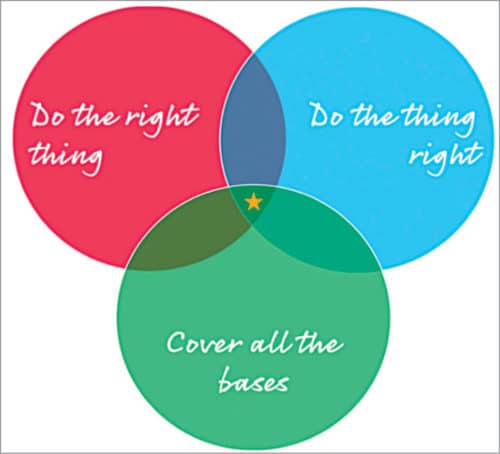
Designing solutions as Responsible AI
While most of the companies understand the need for Responsible AI, they are unclear on how to go about it. Designing solutions as Responsible AI is still a complex and mysterious act.
While researching for my book on AI, Keeping Your AI Under Control, I created a 10-step approach or a framework, The Leash System. This system expertly guides solution development on the correct path, which then would help in designing the solution as Responsible AI. The ten steps are as follows:
- Defining the problem correctly. Define what are you trying to achieve with the AI system, what is your short-term purpose and how does it align with your overall long-term purpose as well as values.
- Establish correct levers or drivers of that problem. Without identifying the right drivers, it is highly challenging to have a correct solution in place.
- Validate issues and its drivers. It is also crucial that by way of simulation or any other mechanism, the problem and its drivers should be validated. Not only that, you will also find it useful to identify which drivers are significant contributors and which ones are not.
- Link AI solution with problem drivers. This advice might sound counter-intuitive, but you see, we don’t solve a problem with solutions, we fix drivers of that problem with solutions, and when we set those drivers, problem automatically gets fixed.
- Verify that fixing key problem-drivers will indeed fix your problem. Sometimes, we come across confounding variables, which appear to be drivers of a problem, but they are not. By validating thoroughly, you can ensure that you are not wasting your efforts and resources that are not fit for AI solution.
- Understand risks. Risks can come in all sizes—small, medium, large. Regardless of that, understanding and acknowledging that it exists is the key.
- Quantify risks. By quantifying risks, you can prioritise your approach towards risk mitigation. And, if not, you will at least be aware of potential issues.
- Establish controls. Once you have identified and quantified risks, establish appropriate control systems, and minimise risks, or better yet eliminate those risks wherever possible.
- The Red Team. It is not anymore just a cybersecurity paradigm. If you want a robust and trustworthy AI system, get your Red team operational.
- Manage residual risk. And finally, despite doing all the above, if anything or any risk remains, manage that residual risk by other possible means.
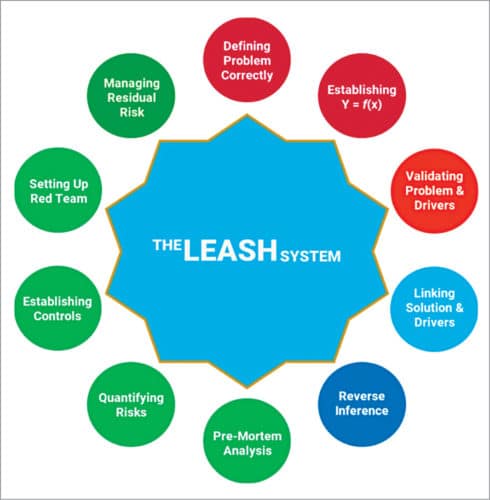
When companies follow this 10-step framework, designing and deploying better AI solutions becomes entirely possible.
Designing responsibility and building trust
However, another critical question remains, “How do we design responsibility and build trust in systems?”
Let’s take real-life analogy here. To trust someone in real life, we typically rely on three things:
- We rely on how someone was taught, their upbringing or education, etc.
- We test them thoroughly to ensure that they have learned it well and understand the rules of society.
- We make sure to provide them with the right operative environment to do their best.
Similarly, for AI to be trustworthy, we must ensure that:
- AI solution is trained well, which means useful data and proper training methods were used. All possible scenarios have been covered, and training lasted for a reasonable duration.
- AI systems are thoroughly tested before deployment, and we keep checking them regularly.
- Things surrounding the AI system are also well integrated with it because, sometimes, the AI system may be good enough, but other interactive systems may throw it off.
By covering these three aspects, I believe we can have reasonable level of trust in an AI system.
Governing automated decision systems
What about the automated decision systems, especially the ones that may have a profound impact on social or personal life? How can we oversee such systems?
I think the best way to implement governance for any automated decision-making system is by making it follow the fundamental rules of the law.
The rule of law says that decision-makers must be transparent and accountable—make an AI system transparent and fix accountability.
The rule of law also says that the decision-maker must be predictable and consistent—make sure that the AI system is predictable and consistent, too.
And finally, the rule of law also says that as a decision-maker, you must always put equality before the law—so, the AI system must be free of bias and discrimination, even if it means diverging from some of the set rules in that case.
In general, I strongly feel the need to have a responsible human being always in the loop when crucial decision making is required. An automated decision system should always recommend not to decide.
Responsible AI practices
There are five practices that each company can follow to ensure the responsible use of AI systems.
- Keeping a balanced scorecard for AI systems’ training and monitoring or say governance. When you have several metrics in a stable scorecard form, you can easily understand how they interact with each other. How maximising or minimising one metric can affect other parameters, and so on.
- Keeping humans in the loop, always. Technology systems may know how to achieve a goal, but they lack common sense, they lack human-level reasoning. So, unless you have a responsible adult who is in charge of this juvenile AI system, there are many ways it could go wrong.
- Keeping a close eye on inputs. There is a reason why we say—garbage in garbage out. If your data has flaws, your AI system will manifest them in output, but at scale. Minor flaws would get amplified to cause problems. There are a few characteristics of a unique full-spectrum dataset—it is timely, complete, correct, integral, accurate, appropriate balance, and it is sufficient. So ensuring that full-spectrum data is available is the key.
- Knowing its limits. AI systems are not all in one solution. Even narrow AI solutions have limitations; they have asymptotic points, that is, situations where the system fails to function. By understanding those limits, everyone can avoid them or manage them effectively.
- Testing thoroughly and keep doing it regularly. First of all, you should always check your AI system for all the potential scenarios. Even if they are only remotely possible or are long-tail cases—testing the system for those cases will ensure that everything is in order.
I often talk about ‘Concept Drift.’ It is the phenomenon where the machine learning model characteristics can change over some time. It means, if you trained and built your system by referring to a particular dataset, it may no longer be relevant. With the change in time and several external factors, the model may be invalid or suboptimal. It is the best practice to test your AI system for concept drift, regularly.
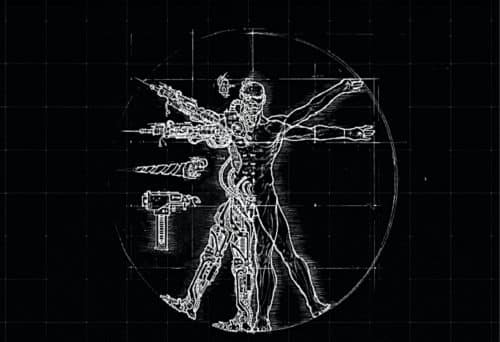
Business challenges in ensuring the responsible use of AI
Many of the businesses are confused as to what to do when it comes to Responsible AI. Simply because there are too many people out there advising companies with Responsible AI and Ethical AI frameworks. The problem with most of them is a conflict of interest. When there is such a conflict, i.e., you are advising and providing AI solutions, too, it rarely yields positive results.
At the same time, many businesses are not even there yet. They are merely ensuring that best practices are followed; they are using standard protocols or frameworks and are maintaining project management hygiene. The issue is that people haven’t understood the vastness, and the scope of the risk when AI goes wrong.
The fact that we don’t know what we don’t know is a significant impediment. It is restricting companies from visualising this problem.
Unlocking the full potential of the workforce
While we take care of risks and other issues related to Responsible AI, how do we unlock the full potential of the workforce?
It is a relatively sensitive issue. There is constant unrest amongst the workforce in several companies. The concern of losing jobs is real and challenging.
However, first, I would recommend that companies should use the AI system only in places where the workforce is complaining about hard work. Whatever your workforce is happily doing, why touch it?
Second, you can use AI solutions, where the time is an essence, and adding more members to the workforce is not a solution. Millisecond decisions or similar ones are good candidates for automation with AI.
Third, use AI solutions where unpredictability is costing more or not bringing more revenue. For example, use it for top-line improvement. If you focus on bottom-line improvement, it will only give measurable efficiency gains.
And for everywhere else, I don’t see an immediate need for an AI solution.
Several years ago, I was working with a company on a time-saving project and even after three months in, we did not achieve any headway. I wasn’t clear what were we missing until one day, one of the colleagues commented – he said, “Even if you give us two hours back in the day, what will we do with it? And because that question is not being answered, we will end up consuming that time in just expanding our existing work.”
That was a light bulb moment; we realised that while we were freeing up their time, we must also ensure that their free time is repurposed for something else, something better.
On the same note, I believe that people are not afraid of losing jobs as such. They are uncomfortable because they do not know what to do with excess time at hand—it bothers them!
So, the best way to unlock the full potential of the workforce would be to gift each of your employees one of the AI bots, like a personal AI assistant, that can do their grunt work.
Then, enable your employees to control those systems effectively and allow them to spend the rest of their time ideating or working strategically.
You can encourage their creativity by taking away mundane and grunt workload. And then provide autonomy to come up with ideas, and also offer them with testbed to validate those ideas.
Responsible AI versus Explainable AI
Some people often confuse Responsible AI with Explainable AI. So, let me give an analogy here. Explainable AI is much like Air Crash Investigation, which is necessary and useful after the fact, but of no use at all to avoid that accident.
Responsible AI is about avoiding that crash in the first place.
When we talk about explainable AI, it is still about being technically thorough. It is about being able to understand what happened, how it happened, and why it happened. And, having an auditable system in place, where you can investigate, after the fact, find out how every step was carried out.
Responsible AI, on the contrary, is being ethically, socially, and morally thorough. It is about being able to control desires, put them second by placing human and societal interest first.
Both are indeed necessary and have an essential place in the ecosystem.
The case for Responsible AI is stronger
Whenever technology intersects with humans—it calls for attention. Not only attention but ethical and responsible approaches.
In the last couple of years, I realised that there is a lot of AI hyperbole. And, this hyperbole has given rise to ‘AI solutionism’ mindset. This mindset makes people believe that if you give them enough data, their machine learning algorithms can solve all of humanity’s problems. People think that throwing more data is the answer, the quality of data is not essential.
We already have seen the rise of a similar mindset a few years ago – something that I would call as “there is an app for it” mindset. We know that it hasn’t done any good in real life to anyone.
Instead of supporting any progress, this type of mindset usually endangers the value of emerging technologies, and it also sets unrealistic expectations. AI solutionism has also led to a reckless push to use AI for anything and everything. And because of this push, many companies have taken a fire-aim-ready approach. It is not only detrimental to the company’s growth, it is also dangerous for customers on many levels.
A better approach would be to consider suitability, applicability, and apply some sanity, do what is necessary. However, this fear of missing out (FOMO) is leading to several missteps, and that is, in-turn creating a substantial intellectual debt, where we do first and think later.
Fear of missing out often creates substantial intellectual debt.
One of the many ways to handle this is by being responsible with technology, especially with AI.
The problem with the Responsible AI paradigm is that everyone knows ‘why’ it is necessary, but no one knows ‘how’ to do it. That prompted me to write a book, Keeping Your AI Under Control.
In this book, I explain ‘how’ part of the Responsible AI. If companies agree that Responsible AI is necessary, this book will guide them on how to achieve it.
I also talk about AI insurance in the book, where, in some cases, it would be helpful to provide remediation to some of the effects of stupid AIs out there.
Remember, you can develop and use AI responsibly if you know the risks. But first, you must understand them better and specify to your use case or context.
Anand Tamboli is a serial entrepreneur, speaker, award-winning published author and emerging technology thought leader.