
Object classification and counting are essential for factory automation and devices where different objects are sorted or counted during packaging. Factories producing buttons, chocolates, and similar items require accurate counting for efficient packaging, which is a time-consuming and costly process when done manually.
Implementing an automation system to detect, classify, sort, and count objects can significantly streamline the production process. This project aims to create a cost-effective device for implementing such a factory automation system.
Object classifications are easily achievable using Edge Impulse models. You can distinguish between a man and an animal, a bicycle and other types of vehicles, and so on.
In our previous article, we did the same
Similarly, you can accurately count one type of object among other types.
Initially designed for MCU-level implementation on devices like ESP32 and Arduino Nicla Vision, the project was intended for counting a small area of 120 pixels x 120 pixels, with a relatively small-sized button as the object of interest.
However, it became apparent that even for this small area, the MCUs were inadequate, as the model file itself is approximately 8MB long. Consequently, the project was eventually installed on a Raspberry Pi computer, where it operates seamlessly. Refer to Fig. 1 for an illustration of the author’s working prototype.
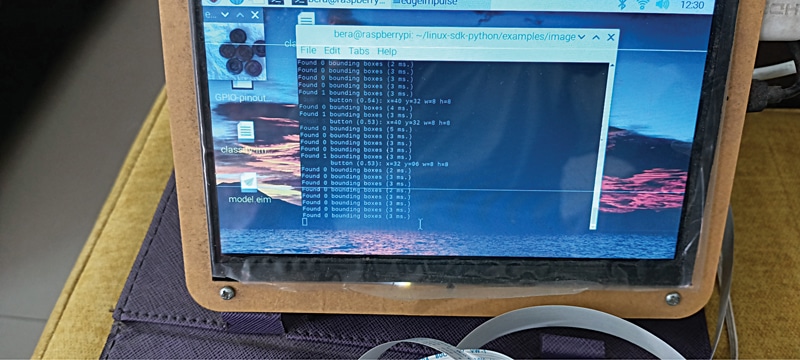
The components required for the project are listed in the Bill of Materials table.
Bill of materials | |
Components | Quantity |
Raspberry PI Zero W /4 | 1 |
RPi camera module | 1 |
SD card 18Gb and above | 1 |
HDMI display | 1 |
Raspberry Pi and Camera Connection
Refer to Fig. 2 for the camera connection with the Raspberry Pi. Connect the Raspberry Pi camera and display as shown in the picture and connect the HDMI display to the HDMI port of the Raspberry Pi.
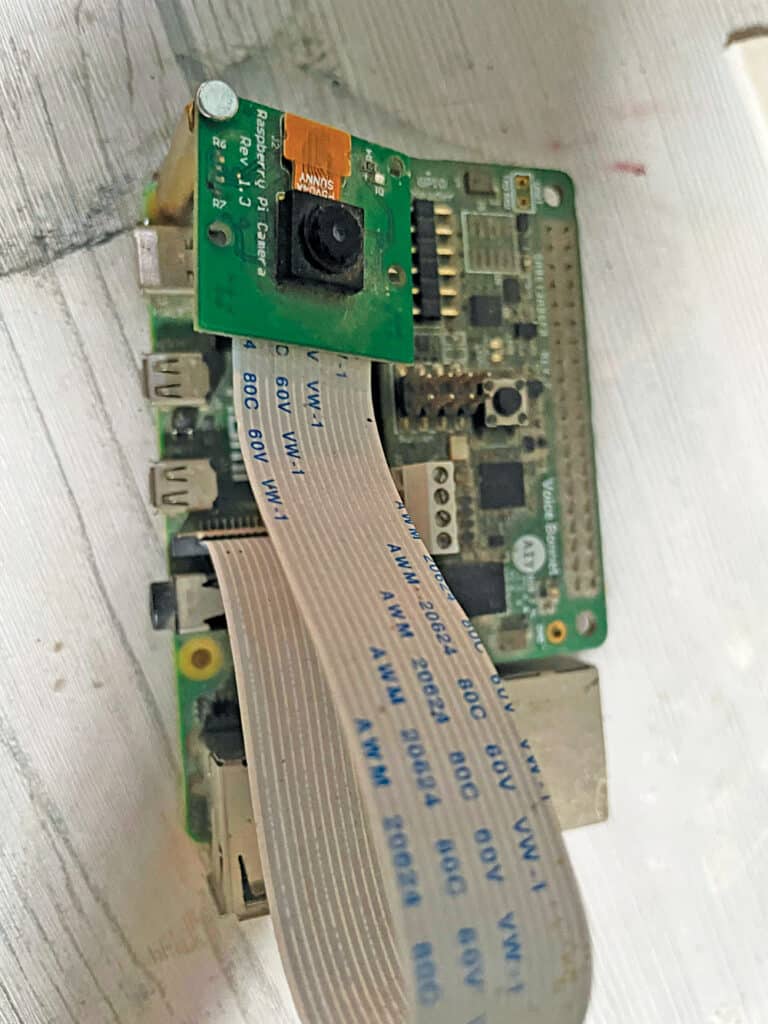
Also Check: Interesting Raspberry Pi Projects
Creating ML Model for Object Classification and Counting
To create the machine language (ML) model, you need to classify and recognize objects. Various platforms like TensorFlow, Edge Impulse, and Google Teachable can be used for this purpose.
Start by opening an account on edgeimpulse.com using an email ID. Collect a handful of similar types of buttons. If you access the site from a Raspberry Pi computer, use the camera to collect images of buttons from various angles, which is crucial for real-world deployment.
Edge Impulse also allows connecting your cell phone or laptop for input device convenience in the data acquisition phase. Refer to Fig. 3 for Edge Impulse device addition and data collection.
The Project
The Edge Impulse project is broadly divided into the following steps:
(a) Data Acquisition: This involves collecting various types of data such as images, sound, temperatures, distances, etc. Some of these data are separated as test data, while all others are used as training data.
(b) Impulse Design: This step is further subdivided into creating impulses, with sub-divisions for:
1. Input parameters: image [width, height], sound [sound parameter].
2. Processing block: How to process the input data
3. Learning block: [Object data of this model]
4. Image processing: Generate features of the collected images
5. Object detection: Select your neural network model and train the model
The object detection part requires expertise, or one could call it a trial-and-error effort, to achieve an accuracy level of 85% or above. There are several models to try, and anything above 90% is considered excellent.
However, it should not be 100% accurate, as this could indicate issues with the data. For this device, the accuracy achieved was 98.6%, which is commendable for a starter project, considering the limited dataset of around 40 instances. Refer to Fig. 3 for more details.
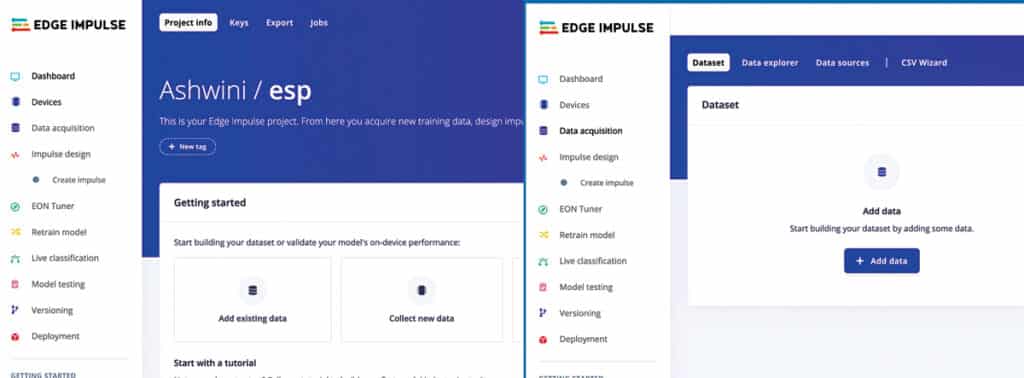
Model Testing
You can test your model on the test data first. See how it works out there, and then point your device to the real-life data to observe its performance! On the browser page, explore this feature. Fig. 4 displays a QR code for testing and running the ML model.
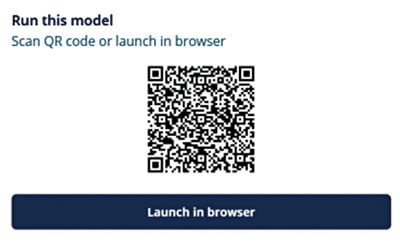
This feature is available in the dashboard of the Edge Impulse opening page. You can scan the image on your mobile and run the model there, or you can run it directly in the browser. Point the camera at the buttons and check whether it can count them. Fig. 5 showcases the data acquisition of three samples.
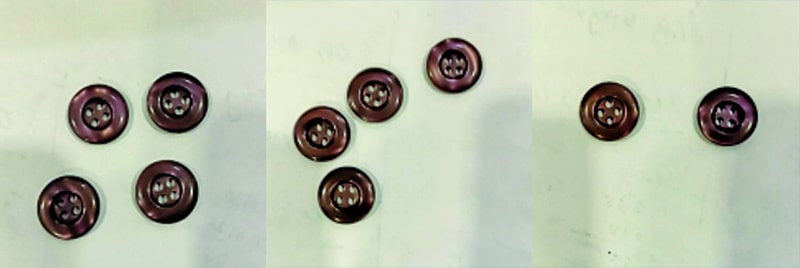
Raspberry Pi – ML Deployment
To run the model on a Raspberry Pi computer, you have to download the *.eim file onto the Raspberry Pi computer. However, unlike other hardware such as Arduino, Nicla Vision, and ESP32, which you can download directly, for Raspberry Pi you need to install Edge Impulse on the Raspberry Pi computer first.
Inside the edge-impulse-daemon software, download this file. Don’t worry; Edge Impulse has a dedicated page for installing Edge Impulse on Raspberry Pi. (Edge Impulse Guide)
Now that Edge Impulse is installed on the Raspberry Pi computer, run edge-impulse-linux-runner.
Note: Keep the internet On for the Raspberry Pi. On a Raspberry Pi computer terminal, connect it to your Edge Impulse page (run edge impulse-linux-runner – clean to switch projects). This command will automatically compile and download the AI model of your device and then start running on your Raspberry Pi computer. (See command.) Show the buttons to the camera connected to your Raspberry Pi, and it will count them.
Deploy Model in Python
In the above deployment, it would work as intended in the Edge Impulse model. To make it work for your specific purpose, such as raising an audio alarm or lighting an LED when the count reaches +2, you have to find some other means. Python3 comes to your aid.
Now, Linux-sdk-python needs to be installed on your Raspberry Pi computer. Edge Impulse SDK (software development kit) is available for various models, such as Python, Node.js, C++, etc. The link below guides you to the page for SDK Python. (SDK Python Guide)
Once Linux-SDK-Python is installed, go to the linux-sdk-python/examples/image directory and run the Python file for image identification.
In the example directory, there are three subdirectories—one each for audio data, image data, and custom data. In the image directory, the video classification file is also available for video input data. The custom directory is for customizing other kinds of data (for experts’ areas only!).
$> python3 classify-image.py /home/bera/downloads/model.eim
That’s how you load the Python file with the downloaded model.eim file. The program will automatically find the camera module (USB connected, or Cam-Port connected) and will start running!
On the top left corner, a small 120×120 camera window will open, and the identified buttons will be marked with a small spot! The numbers identified will be shown on the terminal.
Please ensure sufficient light is available, and the camera is properly focused on the buttons. This is particularly important for cheap cameras. That is why if you run the model on your smartphone, it produces far superior images and counts more quickly. Nonetheless, ensure proper light and focus; it will also produce satisfactory results.
Customize your Model
Examine the classify-image.py file; it is a simple Python file that can be tailor-made with little understanding. In this Python file, we have added the espeak module so that the moment it finds the button(s), it speaks out the number of buttons it finds.
Let’s see part of the Python file to work around:
#!/usr/bin/env python
import device_patches # Device specific
patches – taken care of by the software
import cv2 #import Computer Vision
import os
import sys, getopt
import signal
import time
from edge_impulse_linux.image import
ImageImpulseRunner
import subprocess #this one has been
added by author.
elif “bounding_boxes” in res[“result”].
keys():
print(‘Found %d bounding boxes (%d ms.)’
% (len(res[“result”][“bounding_boxes”]),
res[‘timing’][‘dsp’] + res[‘timing’]
[‘classification’]))
if (len(res[“result”][“bounding_
boxes”])>0):
exitCode = subprocess.call([“espeak”,
”-ven+f3”,”-a200”,” Found %d Buttons” %
len(res[“result”][“bounding_boxes”]) ])
#This one has been added by author.
Epseak is a standalone text-to-speech module for Python. It does not require the Internet to work.
Modified Run
Now you have modified the Python program. If you run the Python file now, it will locate the button [on the top left, a small 120×120 camera port will open], and the numbers will be shown on the terminal window, and the associated speaker will speak out the number—Found five buttons/Found 2 buttons, etc.
If you want to run some relay or light an LED, import the GPIO library of Python, and then fire the associated GPIO to run the relay. However, for running a relay, you have to use a switching transistor to increase the amount of current required for running the relay!
Aftermath
Edge Impulse computing started in 2019 with an objective to enable developers to create the next generation of intelligent devices. The AI-powered programs/devices started appearing on ESP32, Jetson Nano, Raspberry Pi, Orange Pi, Maixduino, OpenMV, Nicla Vision, and many more. This trend will further improve over the coming days! Gone are the days of supercomputers or big-brand, big-sized computers. Small, low-powered modular devices will cover that space in the future.
Somnath Bera is an electronics enthusiast. He is a freelancer and has published several articles across the globe