In the history of life on earth, animals gained the gift of organic vision approximately 700 million years ago. Over time, we were able to use technology to invent the camera and machine vision, with functions similar to human eyes. To use these visuals and take decisions based on various situations in which things could get involved needs higher intelligence.
With the Internet of Things (IoT), it is now possible to bring in awareness to such digital eyes and keep ourselves informed about something out of the ordinary or unfamiliar happening around us. Uncanny Vision, a startup founded by Ranjith Parakkal and Navaneethan Sundaramoorthy, has a product revolving around this innovative idea of teaching the IoT device to identify objects using an analytical system powered by deep learning and smart algorithms. At the grassroots level, the system needs to be taught about various images and visuals that it needs to familiarise with for it to understand how these normally appear. In essence, it is like teaching a child about the world and correcting the perception in order to set proper benchmarks.
Learning the unfamiliar or uncanny
In the biological world, vision is crucial for survival. Visual data helps us make better decisions on various activities. Take the example of a surveillance camera designed to watch over a specific place frequently visited by people on the street. The camera seems to have no other objective apart from staring at a fixed point-of-interest. In the case of a mishap, what if this camera could trigger an important push notification to the nearest police station and help the police arrive in a jiffy? Now that could only be accomplished if an extra awareness feature is added to the set-up so that it can carry out better surveillance while simplifying it for the human involved in decision making.
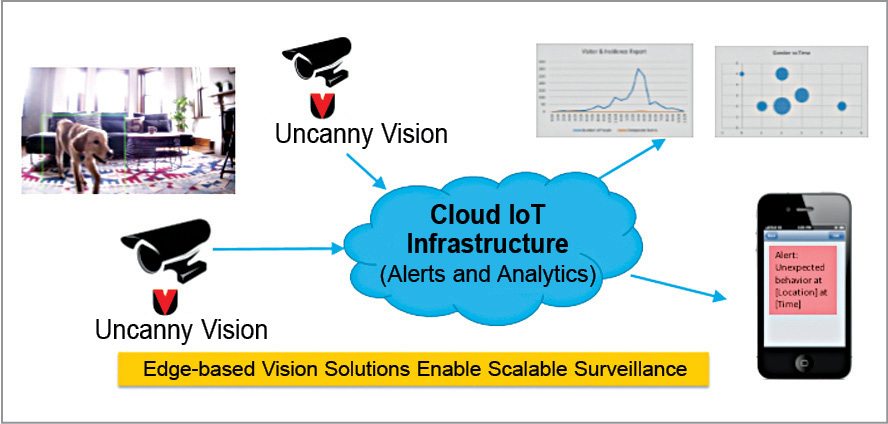
Uncanny Vision works on two prime areas. One is on the edge, which is at the user’s end and the other on the IoT-Cloud infrastructure. This combination is very powerful when it comes to making human-like decisions. The whole system is like an intelligent being that can recognise anything it is programmed to see—even a moving object.
When we say programmed to see, there are a couple of ways that this can be achieved. One way is to train a model (neural network) with thousands of labelled images of target objects that you would like the camera to recognise, for example, gun, helmet, people or wallet. This trained model is optimised and programmed onto the camera hardware. The camera can then recognise objects with reasonable accuracy.
Another way is to have a self-learning camera. In this scenario, the camera (pre-loaded with an untrained model) is given a certain amount of video data and is told that everything in that sample data is normal behaviour. The model learns the definition of normal with the given sample data. The camera is then switched to surveillance mode. It watches and flags any behaviour that it has not seen before, as an anomaly.
An excellent application of this would be to track human movement in an automated teller machine (ATM). These places are often visited by people to swipe cards and get cash out. It might not look like a fast-moving assembly line, but on any given day thousands of people visit ATMs. Let us take an example. If you approach an ATM and do not stand in the position that the system normally recognises, it flags it as an anomaly or unfamiliar sighting.
Smart surveillance is achieved here by giving intelligent sight to the camera itself. If you normally withdraw money and leave, the system does not trigger an anomaly, but if you try to reach out to the ATM in an unfamiliar way, the analytical system evaluates the events based on a scale, which spans from red to green.
Any normal human activity that is expected, for example, standing or walking, would be in the green zone, whereas an unfamiliar or unexpected activity such as you falling down, crouching down or with arms raised up above the head would be in the red zone.
When the system realises that an activity has triggered the red zone, an alert is sent to the responsible personnel or emergency system that it has been programmed to contact. The system can also be taught to detect unauthorised objects that people wear such as helmets or headscarves for early detection of normal behaviour.
Algorithms that learn and adapt
Deep learning may sound fancy but it forms the heart of the system. Uncanny Vision’s computer vision has 70-plus algorithms that cover everything from pedestrian detection, vehicle detection, histograms or holography estimation, and is faster than OpenCV (an open source software used for image processing).
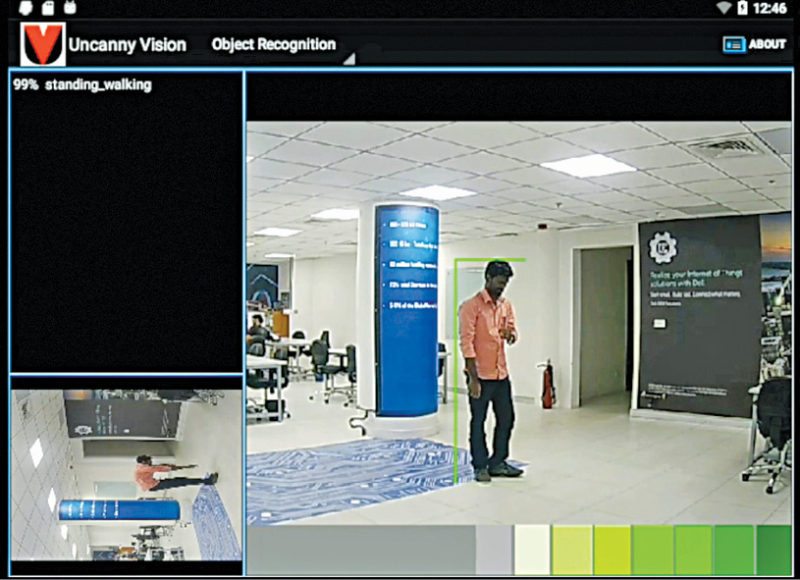
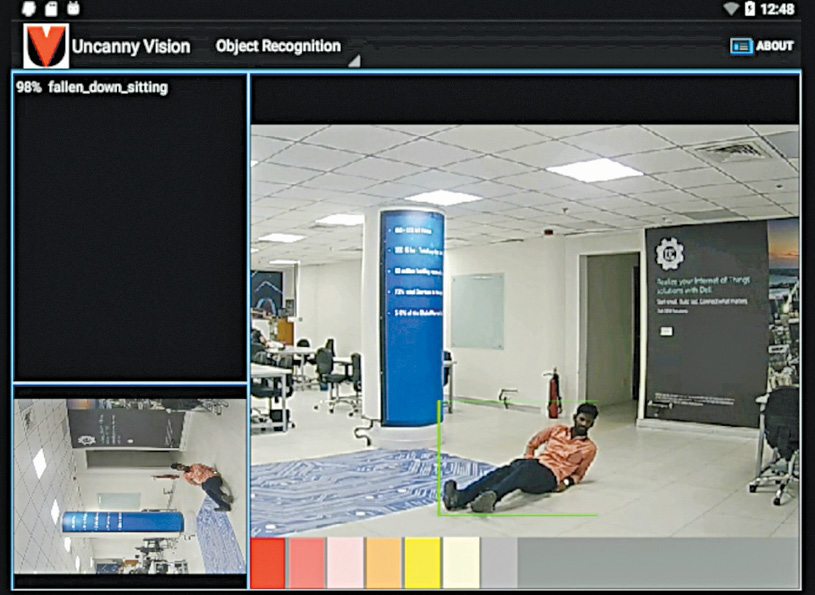
Computer vision in Uncanny Vision is targeted to and optimised for an ARM processor. A new set of cameras that Uncanny works on is similar to the ones used in smartphones as these are equipped with graphic processors and other processing cores for fulfilling all hardware requirements for evaluating the visual sequences captured by these.
Evaluating visual sequences
Processing of data starts after the images reach the CMOS sensor on the camera. From here, these are taken to an image signal processor that cleans up the image from the sensor and then hands it to the main application running on the CPU. All of this happens inside a system-on-chip like Qualcomm’s Snapdragon chips used in most smartphones these days.
This is where Uncanny Vision’s software takes over and interprets the image using deep learning algorithms and convolutional neural network models. These convolutional neural network software models are able to recognise various useful information like human actions and faces from video input, and provide the analytics needed for a surveillance system.
The convolutional neural network models are first trained on GPU based Cloud computers using a tool called Caffe with labelled sample data. Uncanny Vision’s UncannyDL software uses these models in the camera where the hardware equipped with CPU, GPU and brewed models inside the endpoint device detects any anomalies, and pushes out a photo, video, metadata or a decision to the central Cloud platform.
Caffe is a deep learning framework made with expression, speed and modularity. Uncanny Vision uses Caffe for training convolutional neural network models with maximum accuracy and specificity of whatever it sees and identifies. Once the images from video footages are in, deep learning Caffe models kick in and refer to 1000 or more objects it has been taught to recognise. The system can also be taught to recognise animals, human poses and defects.
For machines, deep learning has just began
It may sound like science fiction, but in its simplistic form, deep learning is a sub-field of machine learning that works on the learning levels of representations. Representation can be of any form of digital data in form of images, audio, text and the like. For machines to better understand this part, an artificial neural network could be employed to identify physical objects in a multi-level hierarchy of features, factors and concepts.
A convolutional neural network is a kind of deep neural network in the deep learning concept used in this innovation. It has the capability to study and identify various objects concurrently.
Uncanny Vision is mimicking ways in which human vision and thoughts work through its IoT-Cloud platform for identifying anomalies rather than performing a direct image-to-image match for decision-making. Using this technology, it could be proved that such systems could be used to make our lives easier and secure.
Use cases in retail surveillance and analytics
Retail space has some interesting use cases for visual analytics based IoT systems. Visual data could be used to track movement of people across the display unit in the store and create heat-maps, paths or position-tracking. Path tracking could help a store manager to know the places where more people would possibly frequent and create a heat-map.
Apart from those, reactions and interests towards specific products could also be studied. This would sound advantageous for the retailer as he or she can position the products giving better visibility and thereby maximising probability of the customer buying the right product. The system could be taught to track multiple people and objects just in case there is a huge rush in the store.
Being informed
Uncanny Vision’s IoT-Cloud infrastructure is wired to send out decisions and opinions in the form of notifications to a human with the available interfaces and media such as emails or push notifications on a handheld mobile device. A phenomenal amount of time is saved using Uncanny Vision as the end point device is intelligent enough to send out only processed decisions to the IoT-Cloud infrastructure, because 90 per cent of the time nothing interesting happens in front of a surveillance camera.
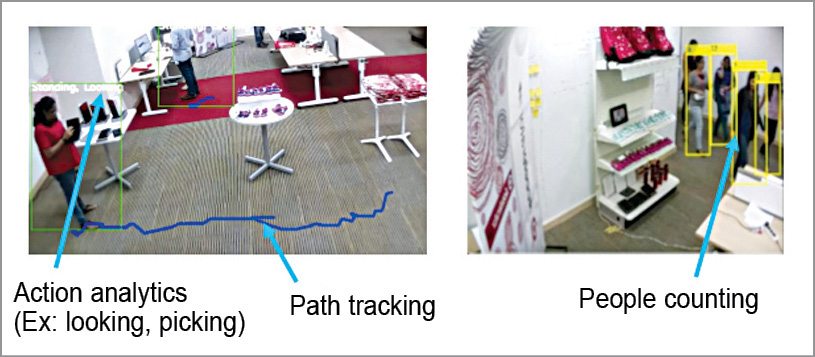
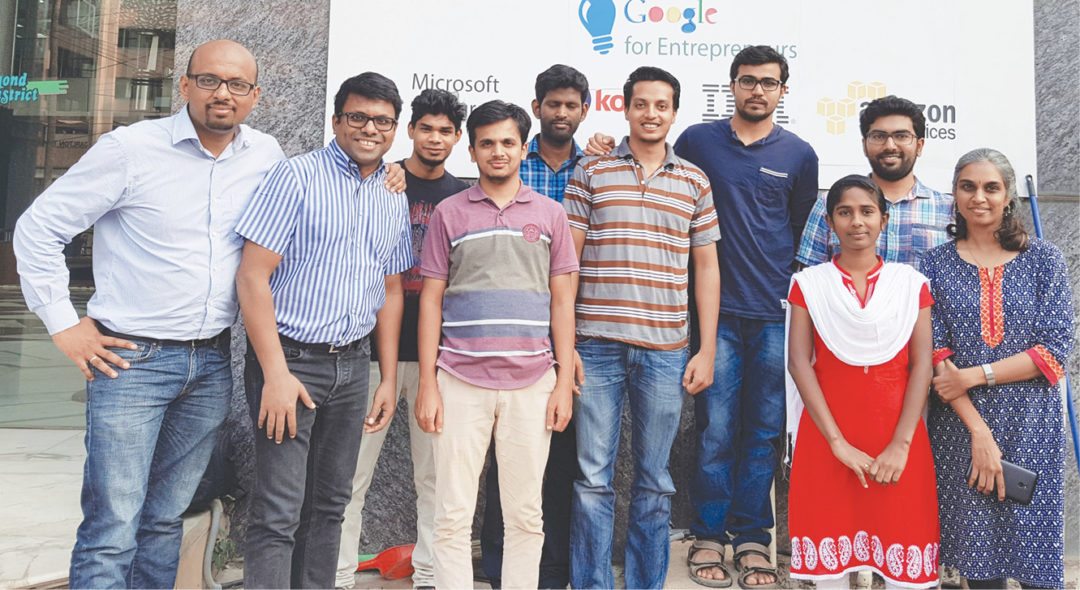
More than just sensing
In surveillance, most of the current 300 million cameras worldwide are blind and can only record video for post-mortem analysis when unexpected incidents happen. In some cases, there are basic analytics that are performed on servers using traditional image-processing algorithms like motion detection in high-security locations, people detection and so on.
Uncanny Vision’s artificial intelligence/deep learning based algorithms can analyse videos a lot deeper and understand what actions humans perform, unexpected visual anomalies and the like.
In addition, Uncanny Vision’s optimised surveillance software can run on high-performance cameras like Qualcomm’s Snapdragon based wireless IP camera and carry out analytics on the device itself, making the system more scalable
Security and surveillance are incomplete without an intelligent system like Uncanny Vision in the days to come. There has been a lot of interest in this field and a multitude of applications are being developed to bring machines to a point of understanding intentions and act in unison with the biological world.
Predictability and machine learning are helping self-driven cars navigate and judge events on the road in real time. Streets could have night-vision-equipped cameras for pointing out crime and eventually eradicating such events.
In a hospital this system could monitor movement of a patient who needs long hours of observation and may need medical attention at will.
Uncanny Vision is more than a vision and can learn more than it can see. It is like having an eye at the back of the head with a mind of its own. Uncanny Vision provides surveillance solutions on a monthly subscription basis, depending on customer requirements. With availability of more data and fine tuning of convolutional neural network models, accuracy of artificial intelligence systems is expected to improve over time.
Shanosh Kumar is technology journalist at EFY. He is BCA from Bangalore University and MBA from Christ University, Bengaluru
can u send me coding of this program please
email: [email protected]
Dear Pankaj, they are not working on open source platforms anymore and have developed their own proprietary softwares.
In the article, there are few open source software mentioned, you can work on them to develop your own.