The article describes how various autopilot applications have evolved and are now helping in making autinomous vehicles that are safe enough to be driven even by the physically challenged and visually impaired people.
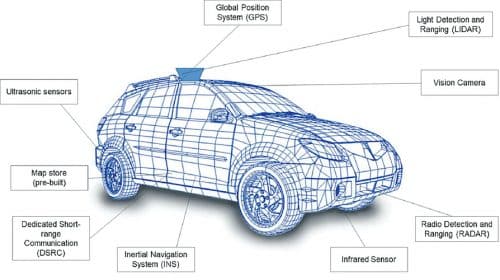
Autonomous vehicles are termed as the ultimate solution for future automotive engineering as these will revolutionise the transportation industry like no other change since the invention of the automobile. For the past hundred years, innovation in automotive sector has created safer, cleaner, and more affordable vehicles, but the progress has been incremental. The industry is now having paradigm shift towards autonomous, self-driving technology. This technology has potential to provide extreme benefits to society in terms of safety on road, no accidents, no congestion, no pollution and, above all, safety and benefits to the disabled persons in the society.
Autonomous vehicle is moving a manual driving vehicle to self-driving using various sensors and actuators that take decision on driving based on various parameters. An autonomous vehicle is capable of environment sensing and operates without any sort of human intervention. A human being is not required to take over the control of the vehicle at any time, nor even to be physically present in the vehicle. A fully automated car is self-aware and capable of making its own decisions.
Autonomous vehicles rely on sensors, actuators, artificial intelligence (AI) based algorithms, and powerful hardware to execute the operations. Autonomous vehicles create and maintain a 3D map of their surroundings based on sensors located in their diverse areas. Radar sensors monitor a vehicle’s position on the road.
Video cameras detect the various objects on road like traffic lights, road signs, vehicles, and even pedestrians. Lidar sensors bounce pulses of light off the car’s surroundings to measure their distances, detect road edges, and identify lane markings. Ultrasonic sensors detect the road and other vehicles in parking areas. AI algorithms process and send instructions to car controls for acceleration, braking, and steering.
Ariel view of autonomous vehicles
Autonomous vehicles are called by many names like robo-car, self-driving vehicle, autonomous car, and driverless car. With technology and sensors for radar, lidar, sonar, GPS, odometer, and inertial measurement unit (IMU), autonomous vehicle can be programmed quickly with auto-pilot capabilities.
Some of the common sensors used in an autonomous vehicle are explained below:
Radar
It uses a sensor that helps to find the range, angle, and velocity of vehicle using radio waves. It is the same technology that is used in spacecraft, guided missiles, and aircraft. It has various transmitters that produce different frequencies of radio waves, which are used to sense various parameters required for vehicle motion.
Lidar
It uses minute laser light emission like a bat that flies and identifies obstacles based on sound reflection. The laser light emitted by lidar is reflected by the obstacles it faces and the reflection time is used to calculate their distance.
Sonar
Sonar is a sound navigation and ranging sensor that uses sound propagation to assist navigation and detection of objects through echo characteristics or acoustic location using very low frequency called infrasonic or very high frequency called ultrasonic. Sonar sensors are of two types: active sonars which emit sound pulses and listen to the echo reflection of the sound and the passive sonars which listen to sound made by different types of objects to identify them.
GPS
Global positioning system (GPS) is a satellite based radio navigation system to get geolocation and time for navigation and potential routes.
Odometer
Odometer uses a technique called Odometry that uses motion sensor data to estimate change in vehicle position and movement relative to a given point or starting location. It is commonly used in robots for leg and hand movements and has inspired use in autonomous vehicle motion.
IMU
Inertial measurement unit is a group of electronic sensors like accelerometer, gyroscope, and magnetometer that is used to measure and report various parameters like force of motion/movement, angular rate, and orientation.
Signage sensor
This is more of an image recognition system to read and interpret signage boards on the roads and feed as input for vehicle movement and direction. For example, signage boards like speed limit and diversion are interpreted and used for vehicle movement, acceleration, and direction.
Companies like Tesla and Waymo have been instrumental in introducing various new sensors and facilities to improve the behaviour of autonomous vehicles. One of the main characteristics of autopilot application is that the software built into the autonomous vehicle has the capability of reprogramming engine so that periodic updates of the software can be provided by vehicle supplier or customised by vehicle owner just like Android updates for smartphones.
One of the key problems in autopilot application and its connected sensors is susceptibility of the sensors and navigation system in specific weather conditions like snow or rainy season and also attack threats like jamming and spoofing. Another challenge can be termed as social challenge where the vehicle has to be updated frequently for high-definition maps, potential future regulations, road condition changes, and route changes.
For precision of vehicle movement and sensor handling, aerial image, high-definition views, and aerial view of sensors like lidar and odometry can help in getting 3D positions for accurate working of autonomous vehicle. For a congested route, aerial imagery maps and lidar using ground reflective position help to get accurate autopilot calculations. This is one of the recent advancements in autonomous vehicle technology that uses the combination of localisation framework of 3D lidar to create local ground reflectivity grid map using aerial localisation (AL) and lidar ground reflectivity (LGR) to enhance the facilities of autopilot applications.
Autopilot applications and their usage
Autopilot is the facility in a vehicle to handle, steer, accelerater, brake automatically based on various inputs from sensors with less or no manual/human inputs. This means we need to design a self-driving car with autopilot software application that should get the inputs from various sensors and actuators and decide how to drive based on these inputs coupled with artificial intelligence based training model in-built in the autopilot application.
Autopilot applications should work in real time to steer or accelerater or apply brake in the vehicle based on inputs received. So the training model and the processing efficiency should be super-fast to be able to provide real-time instructions to control the vehicle. The purpose of autopilot is to reduce manual effort in driving the vehicle and improve automated facilities to do the same. It can be a semi-automated vehicle initially so that training data can be built into the autopilot application. Later it can become fully automated based on trained model and artificial intelligence system built into the application.
Tesla’s autopilot AI
“The advantage that Tesla will have is that we’ll have millions of cars in the field with full autonomy capability and no one else will have that.” — Elon Musk
Tesla is considered as tech leader in the world of electric vehicles. With the integration of artificial intelligence techniques and smart sensors, various features like autopilot are integrated in cars nowadays. Tesla is making use of data science, neural networks, and deep learning for understanding the datasets of customers to gather and predict information about customers and improve the vehicles’ functionalities.
Tesla vehicles have advanced AI features that not only look futuristic but magical in terms of technology. Some of these are described below briefly.
AI integrated chips
Tesla creates AI integrated chips for assistance in lane mapping, autonomous navigation. Six billion transistors are integrated on a single Tesla chip and Tesla chips are 21 times faster than Nvidia chips having 32MB SRAM, which is faster as compared to DRAM.
Autopilot
Tesla integrated traffic-aware cruise control and autosteer features 360-degree visualisation. Tesla uses eight cameras with neural networks to have 360-degree visualisation for safe driving.
Batteries and propulsion system
Tesla uses lithium-ion batteries to pump in more energy and better transmission speeds.
Tesla’s following features make autopilot possible:
- Radar – front facing
- High megapixel cameras – visibility upto 250m
- Digital controlled electric assistance braking system
- Twelve long-range ultrasonic sensors
Cruise control
The radar and forward-facing cameras track the position of cars ahead and adjust the Tesla’s speed accordingly. This feature maintains a safe distance between you and the car in front. The distance between the cars depends on the speed at which both the cars are moving. If a car merges into your lane, the Tesla will monitor its position and reduce speed, if necessary.
Autosteer
The autosteer function lets the Tesla stay centered in a lane, change lanes, and self-park. To keep the Tesla centered, the cameras around the car track the positioning of road markings and the sensors monitor other cars on the road to keep a safe distance. To self-park in parallel and perpendicular positions the car uses both sensors and cameras to avoid hitting any surroundings. The autopilot feature does not work well when the road markings are not clear and/or when the car is traveling under 32kmph. This feature is not recommended for use in residential zones where there are streetlights and stop signs.
Six levels of self-driving autonomy in autopilot applications
The concept of autonomy levels was derived by International Society of Automotive Engineers (SAE) in 2014 under ‘Taxonomy and Definitions for Terms Related to On-Road Motor Vehicle Automated Driving Systems’ report. The report highlighted six levels of self-driving autonomy that auto manufactures need to achieve to attain no-steering wheel technology in the future. Later, in September 2016, the National Highway Traffic Safety Administration (NHTSA) adopted SAE standard.
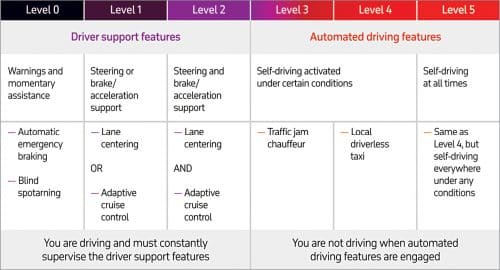
The six levels of self-driving autonomy are:
Level 0: No Automation
It means the human driver is solely responsible for 100% driving task. It is not very hard to understand, and even till today there are modern cars with driver-assistance features and classified as Level 0. The car system can give some warnings like lane departure, forward collision, etc, and even inform the driver via notifications and beep warnings. These vehicles fall under Level 0 category.
Level 1: Driver Assistance
Under Level 1, in certain driving modes, the car can take over the control of steering wheel or pedals, but not both of them at the same time. Example of Level 1 can be adaptive cruise control, where the car will keep a set speed and distance safety with other vehicles on the road and automatically brake if the traffic slows down, and resumes the original speed once normal traffic continues. Another feature is lane assistance, which automatically brings the car to middle position in lane, if vehicle is observed off lane.
Level 2: Partial Automation
In this level, the car can steer, accelerate, and brake in certain conditions. Under this level, the driving task is shared between the vehicle and driver. The vehicle performs two primary driving functions – lateral and longitudinal control by combining adaptive cruise control and lane keeping. In this case, driver can take hands off the vehicle and relax, but still needs to keep a close eye on the surrounding environment.
A new trend under level 2 is Level 2+ Systems, where high degree of automation is observed while entering and existing a highway or lane. Examples: GM’s Super Cruise, Mercedes-Benz Drive Pilot, Tesla Autopilot, Volvo’s Pilot Assistance, and Nissan ProPilot Assist 2.0
Level 3: Conditional Automation
Under conditional automation, the car takes over most of the controls of driving including environmental monitoring. The driver has to pay attention and be ready to take over the control when vehicle prompts. Level 3 automation assists the driver to take off the hands and control from car as long as the driver remains alert. The speed limit where Level 3 conditional automation is recommended is up to 65 kilometres per hour. Example: Audi Traffic Jam Pilot.
Level 4: High Automation
Under this automation, the car can operate without any sort of human assistance but only under selected conditions defined by factors like type of road or geographical area. All the major functionalities like steering, braking, accelerating, and environmental monitoring including lane changing, turning, and signalling can be done by car’s onboard computer. Example: Google’s now-defunct Firefly pod-car prototype, which is restricted to the speed of 40kmph.
Level 5: Full Automation
Level 5 autonomy requires zero human attention. There’s no need for a steering wheel, no need for brakes, and no need for pedals. The autonomous vehicle controls all driving tasks under all conditions, including the monitoring of environment and identification of complex driving conditions like busy pedestrian crossings.
This also means that the vehicle can perform a combination of several tasks simultaneously, whether adaptive cruise control, traffic sign recognition, lane departure warning, emergency braking, pedestrian detection, collision avoidance, cross traffic alert, surround view, park assist, rear collision warning, or park assistance. Example: Waymo project that has yet to design Level 5 tech for self-driving.
Teleoperations and sensors
As per https://www.sae.org/automated-unmanned-vehicles/vehicle automation is classified into five levels (actually six levels in which Level 0 is fully manual driver operation and hence ignoring it). Autonomous vehicle and autopilot application is a complex and contentious technology as it involves a large amount of internal processing using data collected from various sensors and remote operation from outside the line of sight called Teleoperations.
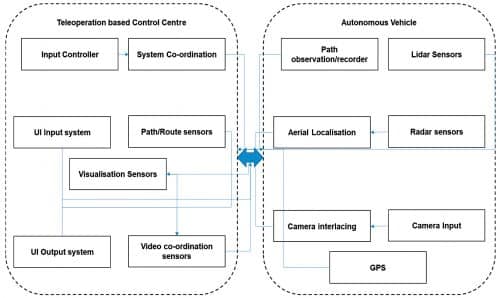
Some of the teleoperations facilities are there for quite some time like anti-lock braking or electronic stability control (ESC), which are used in semi-automatic cars and then have slowly emerged to advanced sensor operations like advanced driver assistance system (ADAS) and crash avoidance system (CAS) which acts quickly during accident times (such as driver protection and alarm sensors). Teleoperation is based on three major controls of autonomous vehicle and its autopilot application design. These are:
Acceleration. To handle the vehicle steering with throttling speed variations based on inputs from obstacles (speed breaker, signage) and angle changes in route.
Direction. Steering action control system to handle left and right movement of the vehicle based on route change and lidar, radar sensor inputs.
Stopping. Brake handling control system to control acceleration and stop the vehicle based on input from different sensors including GPS and IMU.
Teleoperation uses large volume of data and hence does fast processing of data and gives quick commands to control the sensors. Latest advancements like 360° camera view can help in enhancing the autopilot applications for better sensor inputs for vehicle movement and teleoperation activities and control.
So, autopilot application is a revolutionary solution in the automobile industry to create semi-automated and fully-automated vehicles and enable various facilities for physically challenged or visually impaired drivers to control vehicles using voice commands.
Dr Anand Nayyar is PhD in wireless sensor networks and swarms intelligence. He works at Duy Tan University, Vietnam. He loves to explore open source technologies, IoT, cloud computing, deep learning, and cyber security
Dr Magesh Kasthuri is a senior distinguished member of the technical staff and principal consultant at Wipro Ltd. This article expresses his views
and not that of Wipro