Computing-in-memory 3D RRAM devices run complex CNN-based models more energy-efficiently enabling better accuracies and performances.
Machine learning architectures are becoming more complex and computationally demanding. Though machine learning architectures based on convolutional neural networks(CNNs) have proved to be highly valuable in a wide range of applications like computer vision, image processing and human language generation, yet it cannot be applied to a certain level of complex tasks.
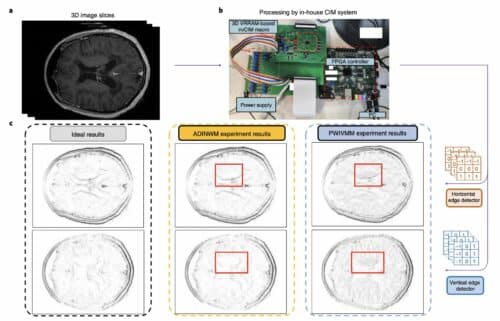
Researchers at the Chinese Academy of Sciences, Beijing Institute of Technology, have recently developed a new computing-in-memory system that could help to run more complex CNN-based models more effectively. This new memory component is based on non-volatile computing-in-memory macros made of 3D memristor arrays.
Resistive random-access memories, or RRAMs, are non-volatile (i.e., retaining data even after breaks in power supply) storage devices based on memristors. Memristors are used to limit or regulate the flow of electrical currents in a circuit, while recording the flow of charge that previously flowed through them.
Embedding the computations inside the memory can greatly reduce the transfer of data between memories and processors, ultimately enhancing the overall system’s energy-efficiency.
This computing-in-memory device created by Qiang Huo and his colleagues is a 3D RRAM with vertically stacked layers and peripheral circuits. The device’s circuit is fabricated using 55nm CMOS technology.
The researchers evaluated their device to run a model for detecting edges in MRI brain scans.”Our macro can perform 3D vector-matrix multiplication operations with an energy efficiency of 8.32 tera-operations per second per watt when the input, weight and output data are 8,9 and 22 bits, respectively, and the bit density is 58.2 bit µm–2,” the researchers wrote in their paper. “We show that the macro offers more accurate brain MRI edge detection and improved inference accuracy on the CIFAR-10 dataset than conventional methods.”
In the future, it could prove to be highly valuable for running complex CNN-based models more energy-efficiently, while also enabling better accuracies and performances.
References: Qiang Huo et al, A computing-in-memory macro based on three-dimensional resistive random-access memory, Nature Electronics (2022).
DOI: 10.1038/s41928-022-00795-x