Researchers make a new approach to trigger atomic movements by applying deep reinforcement learning.
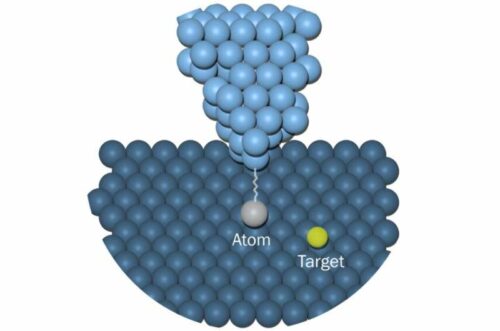
With advancement in the concept of deep learning and neural networks the window of their application is expanding as well. We can apply deep learning algorithms to almost every other computational model and improve its efficiency. And with this study, researchers have expanded the window as they used deep reinforcement learning to steer atoms into a lattice shape, with a view to building new materials or nanodevices.
Researchers used a kind of artificial intelligence called deep reinforcement learning to steer the atoms, each a fraction of a nanometer in size, into the lattice shape. The process is similar to moving marbles around a Chinese checkers board, but with very tiny tweezers grabbing and dragging each atom into place. “We’re also building robotic arms with deep learning, but for moving atoms,” says postdoctoral researcher I-Ju Chen. “Reinforcement learning is successful in things like playing chess or video games, but we’ve applied it to solve technical problems at the nanoscale.”
Researchers explain that such precise movement of atoms is required to design very small devices based on single atoms is important for nanodevices like transistors or memory. Testing how and whether these devices work at their absolute limits is one application for this kind of atomic manipulation, says Chen. Building new materials atom-by-atom, rather than through traditional chemical techniques, may also reveal interesting properties related to superconductivity or quantum states.
The reinforcement part of this type of deep learning refers to how the AI is guided—through rewards for correct actions or outputs. “Give it a goal and it will do it. It can solve problems that humans don’t know how to solve.” Researchers believe that applying machine learning algorithms to nanotechniques can accelerate the parameter selection and trial-and-error usually done by a person.
References : I-Ju Chen et al, Precise atom manipulation through deep reinforcement learning, Nature Communications (2022). DOI: 10.1038/s41467-022-35149-w