Researchers have developed a deep learning method using memristor crossbars enabling error and noise free, high precision computation.
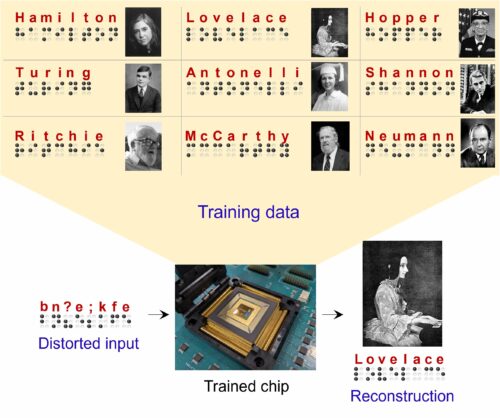
Machine learning models have proven to be highly valuable tools for making predictions and solving real-world tasks that involve the analysis of data. But this can be only possible when they are provided with extensive training which can be both time and energy consuming. Researchers at Texas A&M University, Rain Neuromorphics and Sandia National Laboratories have recently devised a new system for training deep learning models more efficiently and on a larger scale.
This approach could reduce the carbon footprint and financial costs associated with the training of AI models, thus making their large-scale implementation easier and more sustainable. To do this, they had to overcome two key limitations of current AI training practices. The first challenge was the use of inefficient hardware systems based on graphics processing units (GPUs), which are not inherently designed to run and train deep learning models. The second entails the use of ineffective and math-heavy software tools, specifically utilizing the so-called backpropagation algorithm.
The training of deep neural networks entails continuously adapting its configuration, consisting of so-called “weights,” to ensure that it can identify patterns in data with increasing accuracy. This process of adaptation requires numerous multiplications, which conventional digital processors struggle to perform efficiently, as they will need to fetch weight-related information from a separate memory unit.
“As a hardware solution, analog memristor crossbars enable embedding the synaptic weight at the same place where the computing occurs, thereby minimizing data movement. However, traditional backpropagation algorithms, which are suited for high-precision digital hardware, are not compatible with memristor crossbars due to their hardware noise, errors and limited precision.”
Researchers developed a new co-optimized learning algorithm that exploits the hardware parallelism of memristor crossbars. This algorithm, inspired by the differences in neuronal activity observed in neuroscience studies, is tolerant to errors and replicates the brain’s ability to learn even from sparse, poorly defined and “noisy” information.
The brain-inspired and analog-hardware-compatible algorithm was developed to enable the energy-efficient implementation of AI in edge devices with small batteries, thus eliminating the need for large cloud servers that consume vast amounts of electrical power. This could ultimately help to make the large-scale training of deep learning algorithms more affordable and sustainable.