The Researchers from the Hong Kong University of Science and Technology and WeBank developed an effective framework named phonetic-semantic pre- training (PSP) exhibits its strength against synthetic highly noisy speech datasets
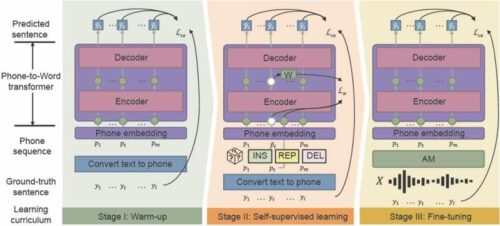
The phonetic-semantic pre-training (PSP) framework assists in recovering misclassified words and excels performance of automatic speech recognition (ASR). The model converts the acoustic model (AM) outputs directly to a sentence with its full context information. Researchers developed a framework that can support the language models (LM) to accurately recover from the noisy outputs of the AM. The PSP framework enables the model to improve through a pretraining regime called a noise-aware curriculum that slowly introduces new skills, initially with easy tasks and then gradually moving to complex tasks.
“Robustness is a long-standing challenge for ASR,” said Xueyang Wu from the Hong Kong University of Science and Technology Department of Computer Science and Engineering. “We want to increase the robustness of the Chinese ASR system with a low cost”. The conventional method trains the acoustic and language models that comprise ASR and requires large amounts of noise-specific data, this results in a costly and time-consuming process. “Traditional learning models are not robust against noisy acoustic model outputs, especially for Chinese polyphonic words with identical pronunciation,” Wu said. “If the first pass of the learning model decoding is incorrect, it is extremely hard for the second pass to make it up.”
The researcher train transducer in two stages: 1) where researchers pre-train a phone-to-word transducer on a clean phone sequence, that is converted from unlabeled text data only to cut back on the annotation time. In this stage, the model initializes the basic parameters to map phone sequences to words. 2) 2nd stage is known to be self-supervised learning, the transducer learns from more complex data produced by self-supervised training techniques and functions. Hence, the resultant phone-to-word transducer is fine-tuned with real-world speech data. The conventional method trains the acoustic and language models that comprise ASR and requires large amounts of noise-specific data, this results in a costly and time-consuming process.
“The most crucial part of our proposed method, Noise-aware Curriculum Learning, simulates the mechanism of how human beings recognize a sentence from noisy speech,” Wu said. The researchers aim to develop more effective PSP pre-training methods with larger unpaired datasets to maximize the effectiveness of pretraining for noise-robust LM.
Click here for the Published Research Paper