Global media has called inventor and author Ray Kurzweil everything from ‘restless genius’ and ‘the ultimate thinking machine’ to ‘rightful heir to Thomas Edison’ and ‘one of the 16 revolutionaries who made America.’ His list of inventions is long—from flat-bed scanners to synthesisers and reading machines for the visually-impaired. He has over 20 honorary doctorates, and has founded a string of successful companies.
There is an interesting story about how this genius, who was not known to work for any company other than his own, joined Google as director of engineering in 2012. He wanted to start a company to build a truly-intelligent computer and knew that no company other than Google had the kind of resources he needed. When he went to meet Larry Page about getting the resources, Page convinced Kurzweil to join them instead. Considering that Google was already well into deep learning research, Kurzweil agreed. It is said that it is the aura of deep learning that closed the deal.
What is this deep learning all about, anyway? It is machine learning at its best—machine learning that tries to mimic the way the brain works, to get closer to the real meaning of artificial intelligence (AI).
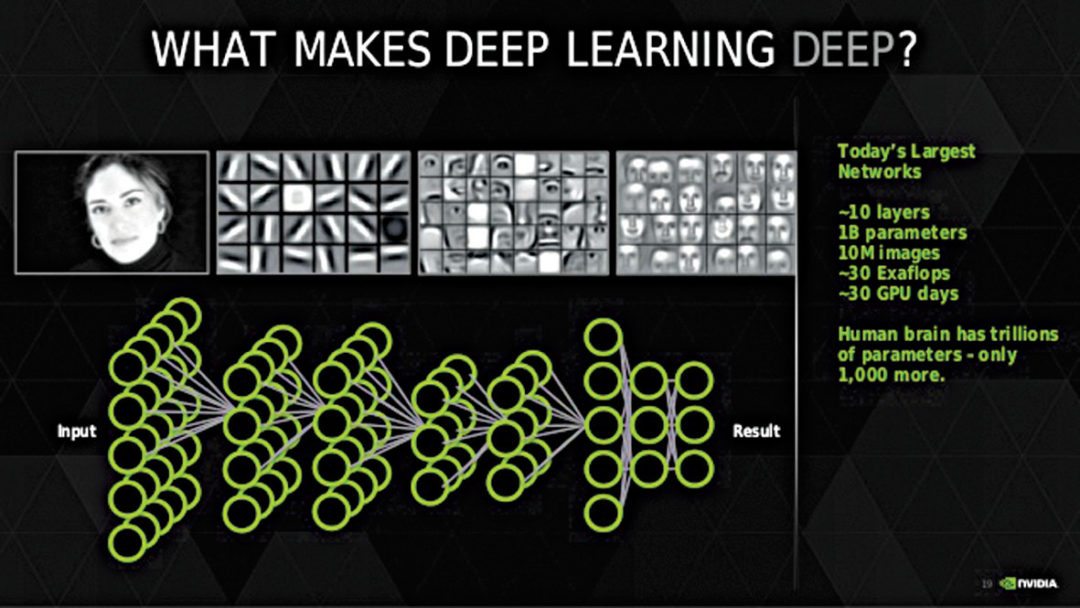
Taking machine learning a bit deeper
Machine learning is all about teaching a machine to do something. Most current methods use a combination of feature extraction and modality-specific machine-learning algorithms, along with thousands of examples, to teach a machine to identify things like handwriting and speech. The process is not as easy as it sounds. It requires a large set of data, heavy computing power and a lot of background work. And, despite tedious efforts, such systems are not fool-proof. These tend to fail in the face of discrepancies. For example, it is easy for a machine-learning system to get confused between a hurriedly written 0 and 6, or vice versa. It can understand brother but not a casually-scribbled bro. How can such machine learning survive in the big, bad, unstructured world?
Deep learning tries to solve these problems and take machine learning one step ahead. A deep learning system will learn by itself, like a child learns to crawl, walk and talk. It is made of multi-layered deep neural networks (DNNs) that mimic the activity of the layers of neurons in the neocortex. Each layer tries to go a little deeper and understand a little more detail.
The first layer learns basic features, like an edge in an image or a particular note of sound. Once it masters this, the next layer attempts to recognise more complex features, like corners or combinations of sounds. Likewise, each layer tries to learn a little more, till the system can reliably recognise objects, faces, words or whatever it is meant to learn.
With the kind of computing power and software prowess available today, it is possible to model many such layers. Systems that learn by themselves are not restricted by what these have been taught to do, so these can identify a lot more objects and sounds, and even make decisions by themselves. A deep learning system, for example, can watch video footage and notify the guard if it spots someone suspicious.
Google has been dabbling with deep learning for many years now. One of its earliest successes was a deep learning system that taught itself to identify cats by watching thousands of unlabelled, untagged images and videos. Today, we find companies ranging from Google and Facebook to IBM and Microsoft experimenting with deep learning solutions for voice recognition, real-time translation, image recognition, security solutions and so on. Most of these work over a Cloud infrastructure, which taps into the computing power tucked away in a large data centre.
As a next step, companies like Apple are trying to figure out if deep learning can be achieved with less computing power. Is it possible to implement, say, a personal assistant that works off your phone rather than rely on the Cloud? We take you through some such interesting deep learning efforts.
From photos to maps, Google uses its Brain
Brain is Google’s deep learning project, and its tech is used in many of Google’s products, ranging from their search engine and voice recognition to email, maps and photos. It helps your Android phone to recognise voice commands, translate foreign language street signs or notice boards into your chosen language and do much more, apart from running the search engine so efficiently.
Google also enables deep learning development through its open source deep learning software stack TensorFlow and Google Cloud Machine Learning (Cloud ML). The Cloud offering is equipped with state-of-the-art machine learning services, a customised neural network based platform and pre-trained models. The platform has powerful application programming interfaces (APIs) for speech recognition, image analysis, text analysis and dynamic translation.
Another of Google’s pets in this space is DeepMind, a British company that it acquired in 2014. DeepMind made big news in 2016, when its AlphaGo program beat the global champion at a game of Go, a Chinese game that is believed to be much more complex than Chess.
Usually, AI systems try to master a game by constructing a search tree covering all possible options. This is impossible in Go—a game that is believed to have more possible combinations than the number of atoms in the universe.
AlphaGo combines an advanced tree search with DNNs. These neural networks take a description of Go board as an input and process it through 12 different network layers containing millions of neuron-like connections. A neural network called the policy network decides on the next move, while another network called the value network predicts the winner of the game.
After learning from over 30 million human moves, the system could predict the human move around 57 per cent of the time. Then, AlphaGo learnt to better these human moves by discovering new strategies using a method called reinforcement learning. Basically, the system played innumerable games between its neural networks and adjusted the connections using a trial-and-error process. Google Cloud provided the extensive computing power needed to achieve this.
What made AlphaGo win at a game that baffled computers till then was the fact that it could figure out the moves and winning strategies by itself, instead of relying on handcrafted rules. This makes it an ideal example of deep learning.
AI world has always used games to prove its mettle, but the same talent can be put to better use. DeepMind is working on systems to tackle problems ranging from climate modelling to disease analysis. Google itself uses a lot of deep learning. According to a statement by Mustafa Suleyman, co-founder of DeepMind, deep learning networks have now replaced 60 handcrafted rule based systems at Google.
Learning from Jeopardy
Some trend-watchers claim that it is Watson, IBM’s AI brainchild, which transformed IBM from a hardware company to a business analytics major. Watson was a path-breaking natural language processing (NLP) computer, which could answer questions asked conversationally. In 2011, it made the headlines by beating two champions at the game of Jeopardy. It was immediately signed on by Cleveland Clinic to synthesise humongous amounts of data to generate evidence based hypotheses, so they could help clinicians and students to diagnose diseases more accurately and plan their treatment better.
Watson is powered by DeepQA, a software architecture for deep content analysis and evidence-based reasoning. Last year, Watson strengthened its deep learning abilities with the acquisition of AlchemyAPI, whose deep learning engines specialise in digging into Big Data to discover important relationships.
In a media report, Steve Gold, vice president of the IBM Watson group, said that, “AlchemyAPI’s technology will be used to help augment Watson’s ability to identify information hierarchies and understand relationships between people, places and things living in that data. This is particularly useful across long-tail domains or other ontologies that are constantly evolving. The technology will also help give Watson more visual features such as the ability to detect, label and extract details from image data.”
IBM is constantly expanding its line of products for deep learning. Using IBM Watson Developer Cloud on Bluemix, anybody can embed Watson’s cognitive technologies into their apps and products. There are APIs for NLP, machine learning and deep learning, which could be used for purposes like medical diagnosis, marketing analysis and more.
APIs like Natural Language Classifier, Personality Insights and Tradeoff Analytics, for example, can help marketers. Data First’s influencer technology platform, Influential, used Watson’s Personality Insights API to scan and sift through social media and identify influencers for their client, KIA Motors. The system looked for influencers who had traits like openness to change, artistic interest and achievement-striving. The resulting campaign was a great success.
Quite recently, IBM and Massachusetts Institute of Technology got into a multi-year partnership to improve AI’s ability to interpret sight and sound as well as humans. Watson is expected to be a key part of this research. In September, IBM also launched a couple of Power8 Linux servers, whose unique selling proposition is their ability to accelerate AI, deep learning and advanced analytics applications. The servers apparently move data five times faster than competing platforms using NVIDIA’s NVLink high-speed interconnect technology.
IBM is also trying to do something more about reducing the amount of computing power and time that deep learning requires. Their Watson Research Center believes that it can reduce these using theoretical chips called resistive processing units or RPUs that combine a central processing unit (CPU) and non-volatile memory. The team claims that such chips can accelerate data speeds exponentially, resulting in systems that can do tasks like natural speech recognition and translation between all world languages.
Currently, neural networks like DeepMind and Watson need to perform billions of tasks in parallel, requiring numerous CPU memory calls. Placing large amounts of resistive random access memory directly onto a CPU would solve this, as such chips can fetch data as quickly as these can process it, thereby reducing neural network training times and power required.
The research paper claims that, “This massively-parallel RPU architecture can achieve acceleration factors of 30,000 compared to state-of-the-art microprocessors—problems that currently require days of training on a data centre size cluster with thousands of machines can be addressed within hours on a single RPU accelerator.” Although these chips are still in the research phase, scientists say that these can be built using regular complementary metal-oxide semiconductor technology.
Locally-done deep learning.
Speaking of reducing processing power and learning times, can you imagine deep learning being performed locally on a mobile phone, without depending on the Cloud? Well, Apple revealed at 2016’s Worldwide Developer’s Conference (WWDC) that it can do precisely that. The company announced that it is applying advanced, deep learning techniques to bring facial recognition to iPhone, and it is all done locally on the device. Some of this success can be attributed to Perceptio, a company that Apple acquired last year. Perceptio is developing deep learning tech that allows smartphones to identify images without relying on external data libraries.
Facebook intends to understand your intent
Facebook Artificial Intelligence Research (FAIR) group has come out with innumerable innovations, which are so deeply woven with their products that we do not even realise that we are using deep learning every time we use Facebook. FbLearner Flow is Facebook’s internal platform for machine learning. It combines several machine learning models to process data points drawn from the activity of the site’s users, and makes predictions such as which user is in a photograph, which post is spam and so on. Algorithms that come out of FbLearner Flow help Facebook to identify faces in photos, select content for your news feed and more.
One of their recent innovations is DeepText, a deep learning based text understanding engine that can understand the textual content posted on Facebook in 20-plus languages. Understanding text might be easy for humans, but for a machine it includes multiple tasks such as classification of a post, recognition of entities, understanding of slang, disambiguation of confusing words and so on.
All this is not possible using traditional NLP methods, and makes deep learning imperative. DeepText uses many DNN architectures, including convolutional and recurrent neural nets to perform word-level and character-level learning. FbLearner Flow and Torch are used for model training.
But, why would Facebook want to understand the text posted by users? Understanding conversations helps to understand intent. For example, if a user says on Messenger that “the food was good at XYZ place,” Facebook understands that he or she is done with the meal, but when someone says, “I am hungry and wondering where to eat,” the system knows the user is looking for a nearby restaurant. Likewise, the system can understand other requirements like the need to buy or sell something, hail a cab, etc. This helps Facebook to present the user with the right tools that solve their problems. Facebook is also trying to build deep learning architectures that learn intent jointly from textual and visual inputs.
Facebook is constantly trying to develop and apply new deep learning technologies. According to a recent blog post, bi-directional recurrent neural nets (BRNNs) show a lot of promise, “as these aim to capture both contextual dependencies between words through recurrence and position-invariant semantics through convolution.” The teams have observed that BRNNs achieve lower error rates (sometimes as low as 20 per cent) compared to regular convolutional or recurrent neural nets for classification.
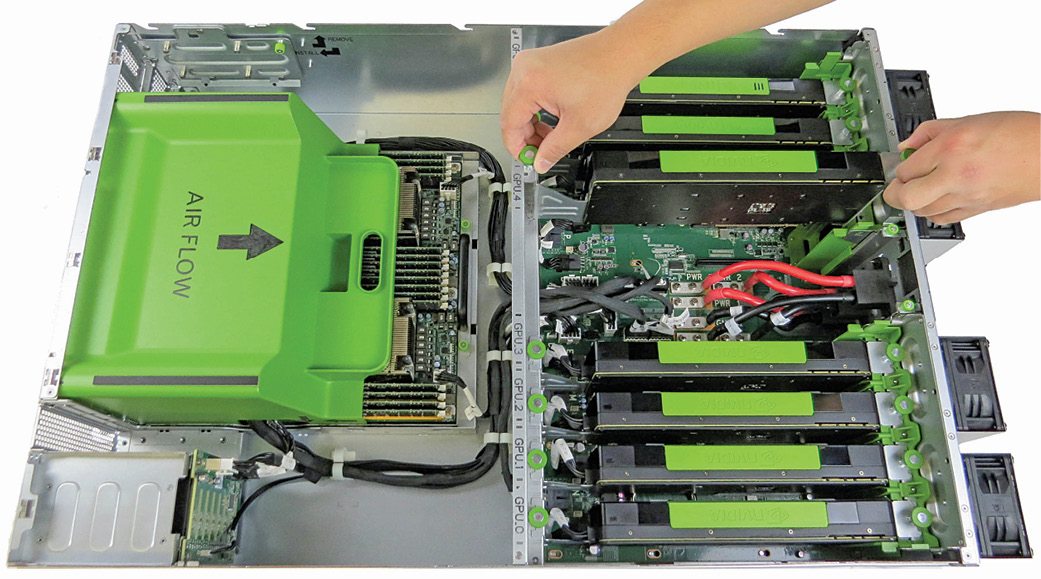
If Google open-sourced its deep learning software engine, Facebook open-sourced its AI hardware last year. Known as Big Sur, this machine was designed in association with Quanta and NVIDIA. It has eight GPU boards, each containing dozens of chips. It has been found that deep learning using GPUs is much more efficient compared to the use of traditional processors. GPUs are power-efficient and help neural nets to analyse more data, faster.
In a media report, Yann LeCun of Facebook said that, open-sourcing Big Sur had many benefits. “If more companies start using the designs, manufacturers can build the machines at a lower cost. And in a larger sense, if more companies use the designs to do more AI work, it helps accelerate the evolution of deep learning as a whole—including software and hardware. So, yes, Facebook is giving away its secrets so that it can better compete with Google—and everyone else.”
Deep learning can deeply impact our lives
Deep learning’s applications range from medical diagnosis to marketing, and we are not kidding you. There is a fabulous line on IBM’s website, which says that we are all experiencing the benefits of deep learning today, in some way or the other, without even realising it.
In June 2016, Ford researchers announced that they had developed a very accurate approach to estimate a moving vehicle’s position within a lane in real time. They achieved this kind of sub-centimetre-level precision by training a DNN, which they call DeepLanes, to process input images from two laterally-mounted down-facing cameras—each recording at an average 100 frames/s.
They trained the neural network on an NVIDIA DIGITS DevBox with cuDNN-accelerated Caffe deep learning framework. NVIDIA DIGITS is an interactive workflow based solution for image classification. NVIDIA’s software development kit has several powerful tools and libraries for developing deep learning frameworks, including Caffe, CNTK, TensorFlow, Theano and Torch.
The life sciences industry uses deep learning extensively for drug discovery, understanding of disease progression and so on. Researchers at The Australian National University, for example, are using deep learning to understand the progression of Parkinson’s disease. In September, researchers at Duke University revealed a method that uses deep learning and light based, holographic scans to spot malaria-infected cells in a simple blood sample, without human intervention.
Abu Qader, a high school student in Chicago, has created GliaLab, a startup that combines AI with the findings of mammograms and fine-needle aspirations to identify and classify breast cancer tumours. The solution starts with mammogram imaging and then sifts Big Data to build predictive models about similar tumour types, risks, growth, treatment outcomes and so on. He used an NVIDIA GeForce GT 750M GPU on his laptop along with TensorFlow deep learning framework.
Deep learning is supposed to be the future of digital personal assistants like Siri, Alexa and Cortana. Bark out any command, and these personal assistants will be able to understand and get it done. Deep learning is also going to be the future of Web search, marketing, product design, life sciences and much more.
Once the Internet of Things (IoT) ensnares the world in its Web, there is going to be Big(ger) Data for deep learning systems to work on. No wonder companies ranging from Google, Facebook, Microsoft and Amazon, to NVIDIA, Apple, AMD and IBM, are all hell-bent on leading the deep learning race. A year down the line, we will have a lot more to talk about!
Janani Gopalakrishnan Vikram is a technically-qualified freelance writer, editor and hands-on mom based in Chennai
The title of the post should be how and what is deep learning. Saying that deeo learning methods make traditional ML look dumb just brings the author’s ignorance in limelight. ML and DL, both are statistical machine learning techniques.
Deep learning is also a statistical machine learning technique, albeit a more radical/ new one. By saying conventional, we were only referring to older ML techniques. There was no intention to put down any technology.