There have been immense and innumerable developments in robotics & AI in recent times—some significant, some not so. Right from form factor and flexibility to motion, sensing and interaction, every aspect of robotics has brought them closer to humans.
Robots are now assisting in healthcare centres, schools, hospitals, industries, war fronts, rescue centres, homes and almost everywhere else. We must acknowledge that this has come about not merely due to mechanical developments, but mainly due to the increasing intelligence, or so-called smartness, of robots.
Smartness is a subjective thing. But in the context of robots, we can say that smartness is a robot’s ability to autonomously or semi-autonomously perceive and understand its environment, learn to do things and respond to situations, and mingle safely with humans. This means that it should be able to think and even decide to a certain extent, like we do.
Let us take you through some assorted developments from around the world that are empowering robots with these capabilities.
Understanding by asking questions
When somebody asks us to fetch something, and we do not really understand which object to fetch or where it is, what do we do? We usually ask questions to zero in on the right object. This is exactly what researchers at Brown University, USA, want their robots to be able to do.
Stefanie Tellex of Humans to Robots Lab of Brown University is using a social approach to improve the accuracy with which robots follow human instructions. The system, called FETCH-POMDP, enables the robots to model their own confusion and solve it by asking relevant questions.
The system can understand gestures, associate these with what the human being is saying and use this to understand instructions better. Only when it is unable to do so does it start asking questions. For example, if you signal at the sink and ask the robot to fetch a bowl, and if there is only one bowl in the sink, it will fetch it without asking any questions. But if it finds more than one bowl there, it might ask questions about the size or colour of the bowl. When testing the system, the researchers expected the robot to respond faster when it had no questions to ask, but it turned out that the intelligent questioning approach managed to be faster and more accurate.
The trials also showed the system to be more intelligent than it was expected to be, because it could even understand complex instructions with lots of prepositions. For example, it could respond accurately when somebody said, “Hand me the spoon to the left of the bowl.” Although such complex phrases were not built into the language model, the robot was able to use intelligent social feedback to figure out the instruction.
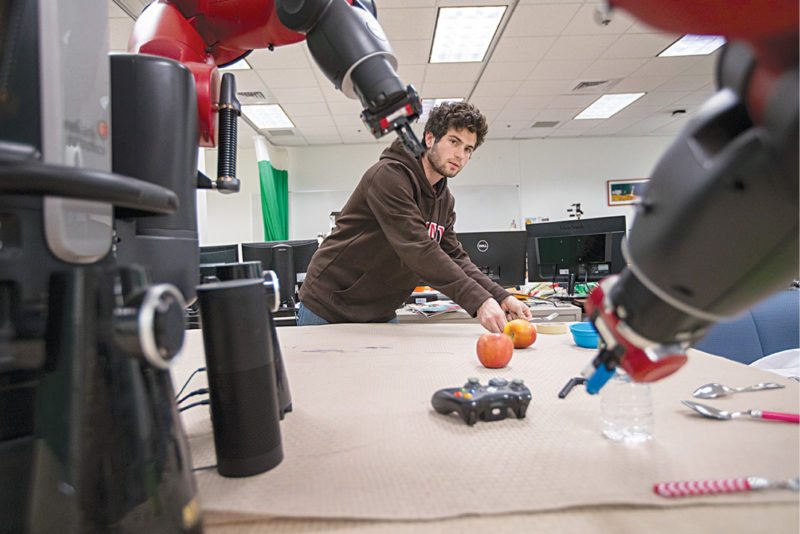
Learning gets deeper and smaller than you thought
Deep learning is an artificial intelligence (AI) technology that is pervading all streams of life ranging from banking to baking. A deep learning system essentially uses neural networks, modelled after the human brain, to learn by itself just like a human child does. It is made of multi-layered deep neural networks that mimic the activities of the layers of neurons in the neocortex. Each layer tries to understand something more than the previous layer, thereby developing a deeper understanding of things. The resulting system is self-learning, which means that it is not restricted by what it has been taught to do. It can react according to the situation and even make decisions by itself.
Deep learning is obviously a very useful tech for robots, too. However, it usually requires large memory banks and runs on huge servers powered by advanced graphics processing units (GPUs). If only deep learning could be achieved in a form factor small enough to embed in a robot!
Micromotes developed at University of Michigan, USA, could be the answer to this challenge. Measuring one cubic millimetre, the micromotes developed by David Blaauw and his colleague Dennis Sylvester are amongst the world’s smallest computers. The duo has developed different variants of micromotes, including smart sensors and radios. Amongst these is a micromote that incorporates a deep learning processor, which can operate a neural network using just 288 microwatts.
There have been earlier attempts to reduce the size and power demands of deep learning using dedicated hardware specially designed to run these algorithms. But so far, nobody has managed to use less than 50 milliwatts of power and the size too has never been this small. Blaauw and team managed to achieve deep learning on a micromote by redesigning the chip architecture, with tweaks such as situating four processing elements within the memory (SRAM) to minimise data movement.
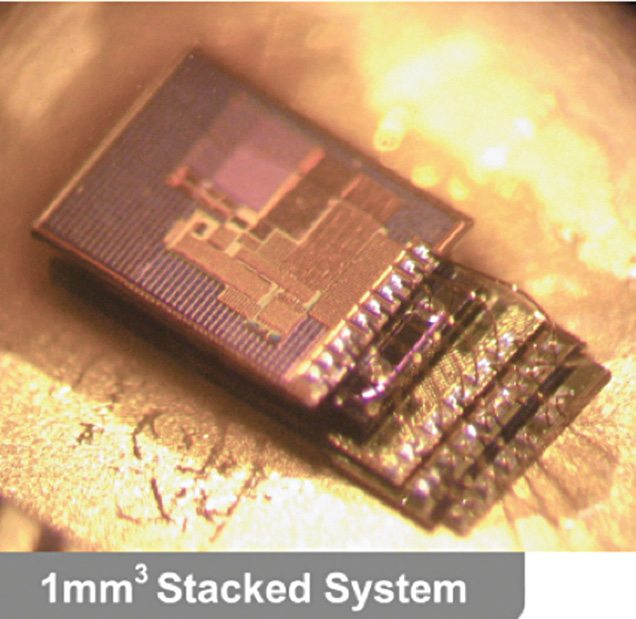
The team’s intention was to bring deep learning to the Internet of Things (IoT), so we can have devices like security cameras with onboard deep learning processors that can instantly differentiate between a branch and a thief lurking on the tree. But the same technology can be very useful for robots, too.
A hardware-agnostic approach to deep learning
Max Versace’s approach to low-power AI for robots is a bit different. Versace’s idea can be traced back to 2010, when NASA approached him and his team with the challenge of developing a software controller for robotic rovers that could autonomously explore planet Mars. What NASA needed was an AI system that could navigate different environments using only images captured by a low-end camera. And this had to be achieved with limited computing, communications and power resources. Plus, the system would have to run on the single GPU chip that the rover had.
Not only did the team manage it, but now Versace’s startup Neurala has an updated prototype of the AI system it developed for NASA, which can be applied for other purposes. The logic is that the same technology that was used by Mars rovers can be used by drones, self-driving cars and robots to recognise objects in their surroundings and make decisions accordingly.
Neurala too bets on deep learning as the future of its AI brain, but unlike most common solutions that run on online services backed by huge servers, Neurala’s AI can operate on the computationally low-power chips found in smartphones.
In a recent press report, Versace hinted that their approach focuses on edge computing, which relies on onboard hardware, in contrast with other approaches that are based on centralised systems. The edge computing approach apparently gives them an edge over others. This is because the key to their system is hardware-agnostic software, which can run on several industry-standard processors including ARM, Nvidia and Intel.
Although their system has already been licensed and adapted by some customers for use in drones and cars, the company is very enthusiastic about its real potential in robot toys and household robots. They hope that their solution will ensure fast and smooth interaction between robots and users, something that Cloud systems cannot always guarantee.
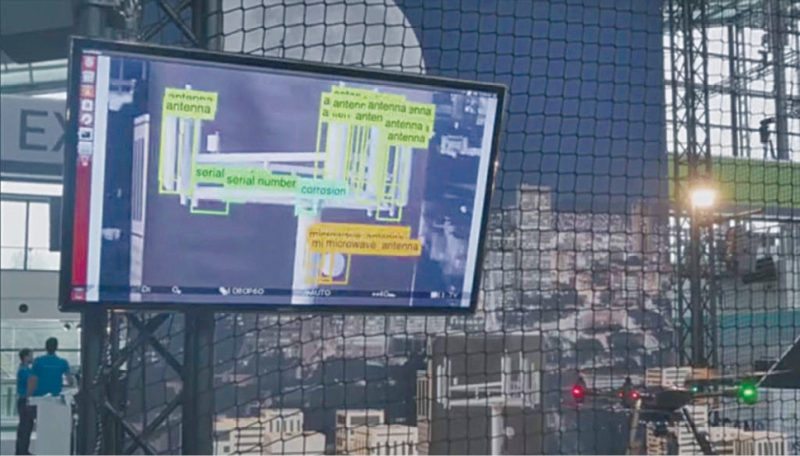
Analogue intelligence, have you given it a thought
Shahin Farshchi, partner at investment firm Lux Capital, has a radically different view of AI and robots. He feels that all modern things need not necessarily be digital, and analogue has a great future in AI and robotics. In an article he wrote last year, he explained that some of the greatest systems were once powered by analogue, but it was abandoned for digital systems just because analogue was rigid and attempting to make it flexible made it more complex and reduced its reliability.
As Moore’s law played its way into our lives, micro-electro-mechanical systems and micro-fabrication techniques became widespread, and the result is what we see all around us. He wrote, “In today’s consumer electronics world, analogue is only used to interface with humans, capturing and producing sounds, images and other sensations. In larger systems, analogue is used to physically turn the wheels and steer rudders on machines that move us in our analogue world. But for most other electronic applications, engineers rush to dump signals into the digital domain whenever they can. The upshot is that the benefits of digital logic—cheap, fast, robust and flexible—have made engineers practically allergic to analogue processing. Now, however, after a long hiatus, Carver Mead’s prediction of the return to analogue is starting to become a reality.”
Farshchi claims that neuromorphic and analogue computing will make a comeback in the fields of AI and robotics. Neural networks and deep learning algorithms that researchers are attempting to implement in robots are more suitable to analogue designs. Such analogue systems will make robots faster, smaller and less power-hungry. Analogue circuits inspired by nature will enable robots to see, hear and learn better while consuming much less power.
He cites the examples of Stanford’s Brains in Silicon project and University of Michigan’s IC Lab, which are building tools to make it easier to build analogue neuromorphic systems. Some startups are also developing analogue systems as an alternative to running deep nets on standard digital circuits. Most of these designs are inspired by our brain, a noisy system that adapts according to the situation to produce the required output. This is in contrast to traditional hard-coded algorithms that go out of control if there is the slightest problem with the circuits running these.
Engineers have also been able to achieve energy savings of the order of 100 times by implementing deep nets in silicon using noisy analogue approaches. This will have a huge impact on the robots of the future, as they will not require external power and will not have to be connected to the Cloud to be smart. In short, the robots will be independent.
Training an army of robots using AI and exoskeleton suits
Kindred is a quiet but promising startup formed by Geordie Rose, one of the co-founders of D-Wave, a quantum computing company. According to an IEEE news report, Kindred is busy developing AI-driven robots that can possibly enable one human worker do the work of four. Their recent US patent application describes a system in which an operator wears a head-mounted display and an exoskeleton suit while doing his tasks. Data from the suit and other external sensors is analysed by computer systems and used to control distant robots.The wearable robotic suit includes head and neck motion sensors, devices to capture arm movements and haptic gloves. The operator can control the robot’s movement using a foot pedal, and see what the robot is seeing using a virtual reality headset. The suit might also contain sensors and devices to capture brain waves.
The robot is described as a humanoid of 1.2-metre height, possibly covered with synthetic skin, with two (or more) arms ending in hands or grippers and wheeled treads for locomotion. It has cameras on its head and other sensors like infrared and ultraviolet imaging, GPS, touch, proximity, strain sensors and radiation detectors to stream data to its operator.
Something that catches everybody’s attention here is a line that says, “An operator may include a non-human animal such as a monkey… and the operator interface may be… re-sized to account for the differences between a human operator and a monkey operator.”
But what is so smart about an operator controlling a robot, even if the operator is a monkey? Well, the interesting part of this technology is that the robots will also, eventually, be able to learn from their operators and carry out the tasks autonomously. According to the patent application, device-control instructions and environment sensor information generated over multiple runs may be used to derive autonomous control information, which may be used to facilitate autonomous behaviour in an autonomous device.
Kindred hopes to do these using deep hierarchical learning algorithms like a conditional deep belief network or a conditional restricted Boltzmann machine, a type of powerful recurrent neural network.
This is what possibly links Kindred to D-Wave. The operation of D-Wave’s quantum computing system is described by these as being analogous to a restricted Boltzmann machine, and its research team is working to exploit the parallels between these architectures to substantially accelerate learning in deep, hierarchical neural networks.
In 2010, Rose also published a paper that shows how a quantum computer can be very effective at machine learning. So if Kindred succeeds in putting two and two together, we can look forward to a new wave of quantum computing in robotics.
Robots get more social
It is a well-known fact that technology can help disabled and vulnerable people to lead more comfortable lives. It can assist them to do their tasks independently without requiring another human being to help them. This improves their self-esteem.
However, Maja Matarić of University of South California, USA, believes that the technology can be more assistive if it is embodied in the form of a robot rather than a tool running on a mobile device or an invisible technology embedded somewhere in the walls or beds.
Matarić’s research has shown that the presence of human-like robots is more effective in getting people to do things, be it getting senior citizens to exercise or encouraging autistic children to interact with their peers. “The social component is the only thing that reliably makes people change behaviour. It makes people lose weight, recover faster and so on. It is possible that screens are actually making us less social. So that is where robotics can make a difference—this fundamental embodiment,” Matarić mentioned while addressing a gathering at American Association for the Advancement of Science.
Matarić is building such robots through her startup Embodied Inc. Research and trials have shown promising results. One study found that autistic children showed more autonomous behaviour upon copying the motions of socially-assistive robots.
In another study, patients recovering from stroke responded more quickly to upper-extremity exercises when prompted and motivated by socially-assistive robots.
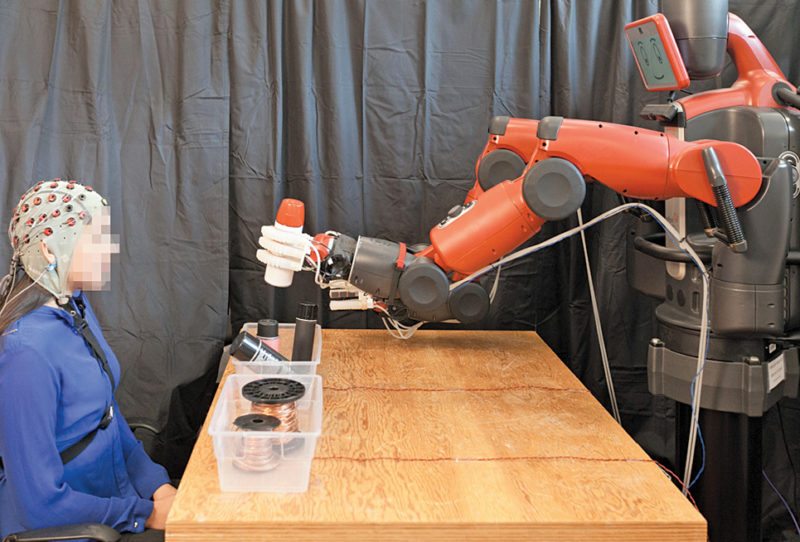
Robots can mingle in crowded places, too
Movement of robots was once considered a mechanical challenge. Now, scientists have realised it has more to do with intelligence. For a robot to move comfortably in a crowded place like a school or an office, it needs to first learn things that we take for granted. It needs to learn the things that populate the space, which of these things are stationary and which ones move, understand that some of these things move only occasionally while others move frequently and suddenly, and so on. In short, it needs to autonomously learn its way around a dynamic environment. This is what a team at KTH Royal Institute of Technology in Stockholm hopes to achieve.
Rosie (yes, we know it sounds familiar) is a robot in their lab that has already learnt to perceive 3D environments, move about and interact safely in these. Rosie repeatedly visits the rooms at the university’s Robotics, Perception and Learning Lab, and maps these in detail. It uses a depth camera (RGB-D) to grab points of physical space and dump these into a database, from which 3D models of the rooms can be generated.According to a news report, “The system KTH researchers use detects objects to learn by modelling the static part of the environment and extracting dynamic elements. It then creates and executes a view plan around a dynamic element to gather additional views for learning. This autonomous learning process enables Rosie to distinguish dynamic elements from static ones and perceive depth and distance.” This helps the robot understand where things are and negotiate physical spaces.
Just a thought can bring the robot back on track
It is one thing for robots to learn to work autonomously, it is another for them to be capable of working with humans. Some consider the latter to be more difficult. To be able to co-exist, robots must be able to move around safely with humans (as in the case of KTH’s Rosie) and also understand what humans want, even when the instruction or plan is not clearly, digitally explained to the robot.
Explaining things in natural language is never foolproof because each person has a different way of communicating. But if only robots could understand what we think, the problem would be entirely solved. As a step towards this, Massachusetts Institute of Technology’s Computer Science and Artificial Intelligence Laboratory (CSAIL) and Boston University are creating a feedback system that lets you correct a robot’s mistakes instantly by simply thinking about it.
The experiment basically involves a humanoid robot called Baxter performing an object-sorting task and a human watching it. The person watching the robot has to wear a special head gear. The system uses an electroencephalography monitor to record the person’s brain activity. A novel machine learning algorithm is applied to this data to classify brain waves in the space of 10 to 30 milliseconds. When the robot indicates its choice, the system helps it to find out whether the human agrees with the choice or notices an error. The person watching the robot does not have to gesture, nod or even blink. He or she simply needs to agree or disagree mentally with the robot’s action. This is much more natural than earlier methods of controlling robots with thoughts.
The team lead by CSAIL director Daniela Rus has managed to achieve this by focusing the system on brain signals called error-related potentials (ErrPs), which are generated whenever our brains notice a mistake. When the robot indicates the choice it is about to make, the system uses ErrPs to understand whether the human supervisor agrees with the decision.
According to the news report, “ErrP signals are extremely faint, which means that the system has to be fine-tuned enough to both classify the signal and incorporate it into the feedback loop for the human operator.”
Additionally, the team has also worked on the possibility of the system not noticing the human’s original correction, which might lead to secondary errors. In such a case, if the robot is not sure about its decision, it can trigger a human response to get a more accurate answer. Further, since ErrP signals appear to be proportional to how bad the mistake is, future systems could be extended to work for more complex multi-choice tasks.
This project, which was partly funded by Boeing and National Science Foundation, can also be useful for physically-challenged people to work with robots.
Calling robots electronic persons, is it a slip or the scary truth
Astro Teller, head of X (formerly Google X), the advanced technology lab of Alphabet, explained in a recent IEEE interview that washing machines, dishwashers, drones, smart cars and the like are robots though these might not be jazzy-looking bipeds. These are intelligent, help us do something and save us time. If you look at it that way, smart robots are really all around us.
It is easy to even build your own robot and make it smart, with simple components and open source tools. Maybe not something that looks like Rosie or Baxter, but you can surely create a quick and easy AI agent. OpenAI Universe, for example, lets you train an AI agent to use a computer like a human does. With Universe, the agent can look at screen pixels and operate a virtual keyboard and mouse. The agent can be trained to do any task that you can achieve using a computer.
Sadly, the garbage-in-garbage-out principle is true for robotics and AI, too. Train it to do something good and it will. Train it to do something bad and it will. No questions asked. Anticipating such misuse, the industry is getting together to regulate the space and implement best practices. One example is Partnership on Artificial Intelligence to Benefit People and Society, comprising companies like Google’s DeepMind division, Amazon, Facebook, IBM and Microsoft. The website speaks of best practices, open engagement and ethics, trustworthiness, reliability, robustness and other relevant issues.The European Parliament, too, put forward a draft report urging the creation and adoption of EU-wide rules to manage the issues arising from the widespread use of robots and AI. The draft helps us understand the need to standardise and regulate the constantly mushrooming variety of robots, ranging from industrial robots, care robots, medical robots, entertainment robots and drones to farming robots.
The report explores the issues of liability, accountability and safety, and raises issues that make us pinch ourselves and understand that yes, we are really co-existing with robots. For example, who will pay when a robot or a self-driving car meets with an accident, when robots will need to be designated as electronic persons, how to ensure they are good ones and so on. The report asserts the need to create a European agency for robotics and AI to support the regulation and legislation efforts, the need to define and classify robots and smart robots, create a robot registration system, improve interoperability and so on.
However, it is the portion about robots being called electronic persons that has raised a lot of eyebrows and caused a lot of buzz among experts. Once personhood is associated with something, issues like ownership, insurance and rights come into play, making the relationship much more complex.
Comfortingly, one of the experts had commented that since we build robots, these are like machine slaves, and we can choose not to build robots that would mind being owned. In the words of Joanna Bryson, a working member of IEEE Ethically Aligned Design project, “We are not obliged to build robots that we end up feeling obliged to.”
When equipped with self-learning capabilities, what if they learn to rebel? Remember how K-2SO swapped sides in Star Wars movie Rogue One? Is there such a thing as trusted autonomy? Well, another day, another discussion!