Artificial intelligence (AI) technology is employed in safety-critical situations such as airports, ATM machines and aircraft operations. When decision-makers and business executives have reliable data analyses, recommendations and follow-ups through AI systems, they can make smarter decisions and better choices for their business, employees
and future actions
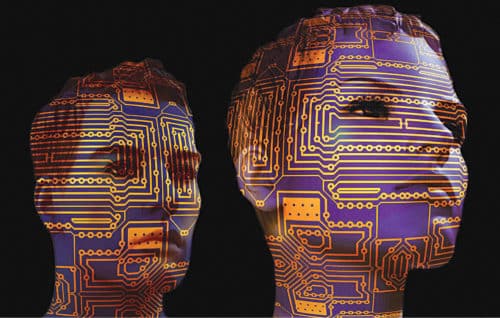
Show a photo of a dog to a three-year-old child, and she or he will be able to tell you it is a dog, and that may not be considered remarkable. But if a machine can tell you the same thing, it would be considered remarkable. This is because for a machine to achieve that feat would require a form of artificial intelligence (AI) development called deep learning.
The machine has to be trained using thousands of dog images, otherwise it will merely perceive it as a blob and give an incorrect answer. In the above example, unlike the machine, the child can learn things intuitively and unsupervised, and it comes as no surprise when she or he can easily recognise the animal.
Not Hotdog app seems farcical and absurd, but it has a very interesting factual and practical aspect to it. This app is based on cutting-edge AI and computer vision technologies that can identify a hotdog from other food items or objects. It was developed for fun and experimentation. But with advancements in modern technology, we can use AI algorithms to easily identify animals, objects, places and people for use in critical applications.
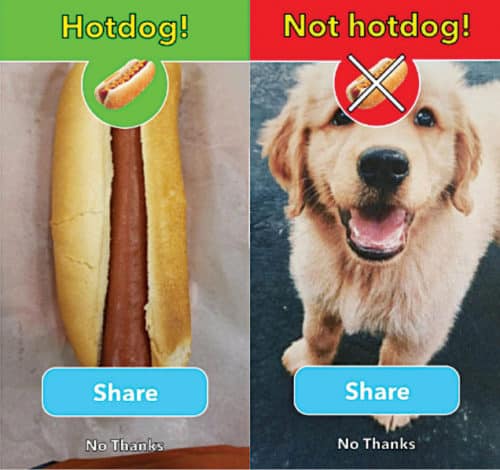
It is easy to build an AI app like Not Hotdog for fun and experimentation, but what does it take to build a mission-critical AI application that you can trust to help run a business or accomplish a task successfully? Let us take a look at a few cases of AI in mission-critical applications in this article.
If Not Hotdog app does not give a correct answer, it will not matter much as it is not critical. But think of a situation where a computer vision system outputs wrong information in skin cancer detection, or a self-driving car is unable to detect an object in front of it, the situation where the visual object detection system fails to detect the enemy in a military operation?
The results would be catastrophic and cause irreparable damage. These are critical applications where accuracy, timing and quality are extremely important.
AI technology related to computer vision, image processing, machine learning and deep learning has many applications across industries.
In consumer electronics, these applications provide face recognition and autofocus to camera-enabled devices.
In the public sector, these can be used to identify dangerous driving situations with traffic monitoring cameras.
In healthcare, these can be used for medical imaging and diagnosis.
In retail, these can be used to spot malicious activities in stores.
In agriculture, these can be used to determine the health of crops in the field.
All of these applications are critical to core businesses and processes.
In critical applications, quality and quantity of input data are important to obtain correct results. Deep learning based on a deep neural network, a class of machine learning algorithm, is important in mission-critical applications. Deep learning uses multiple layers to progressively extract higher-level features from raw data input, enhancing accuracy and giving more reliable results.
Key differences in mission-critical AI
The context and levels of criticality may differ from industry to industry, but there are a few parameters common to all such applications involving AI technology. Mission-critical, time-critical, life-critical and safety-critical applications require fast identification, timely action, data delivery, reliable notification and warning messages. Key differences in mission-critical AI applications are accuracy, service-level agreement (SLA), scalability and security.
Accuracy
Mission-critical AI applications need significant and better accuracy. This means that training data needs to be greater in quality and quantity. Actually, quantity of data you need depends on complexity of algorithms for a particular problem.
SLA
All core business processes have strict SLAs. An SLA is a commitment between a service provider and a client. The commitment includes quality, availability and responsibilities. Services should be provided to the customer as agreed upon in the contract. For example, when an image is fed to a model for prediction, as per requirement, the model should not take much time to predict the correct result. If there is a delay, it is not following a strict SLA.
SLAs may include many components, from a definition of services to termination of agreement.
Scalability
Besides SLAs, architecture has to be scalable. High accuracy and strict SLAs need an architecture that can effortlessly scale for both computing and storage.
Security
This is one of the prime concerns in AI technology. Certain applications present security and data privacy concerns. Data may include sensitive information that has to be stored securely with enterprise-grade security features. In mission-critical AI applications, strict security measures are taken into account and only authorised users have access to information.
AI components in mission-critical applications
Essential components for mission-critical AI applications include datasets and hardware setup. In machine learning, construction of algorithms is done through building a mathematical model from input data.
Datasets
Quantity and quality of data are important when it comes to AI applications. Data used to build the final model usually comes from multiple datasets. In most cases, three datasets are used in different stages of the creation of the model. For any given dataset, there are many possible models to identify them with fundamentally different interpretations.
Input data
This is one the most important components when it comes to AI technology. Imagine there are many cameras installed in a retail store having different outlets. From input data received from these camera sensors, the user may have to process thousands of images per minute to detect malicious activities.
Training data
For mission-critical AI applications, accuracy and quality are important. An AI model is useful if input data is trained on. For example, implications of failing to recognise skin cancer can be fatal. We need datasets that are significantly greater in quantity and quality. This means we may have to spend significant time in analysing, preparing and labelling data.
Feedback
A feedback loop improves the model. For example, in the retail store, it would be a good idea to label false predictions and feed them back to the model for retraining. A continuous feedback loop increases training data size, and compute requirements also increase but with better accuracy and results.
Hardware setup
AI is based on algorithms, but without a hardware setup or platform, algorithms do not make any sense. Unfortunately, the importance of hardware is not discussed or included in most of the materials found on the Internet.
An AI ecosystem contains various types of agents including hardware and software. Hardware includes sensors, processors and storage. Hardware used for running mission-critical applications also depend on SLAs and differences in data set sizes.
Sensors
These detect or measure physical properties, and record, indicate or otherwise respond to them as per input data. For example, in image processing, input data is fed from vision sensors in a camera system. No matter how complex or good the algorithm is, without a good vision sensor the output will not be accurate as expected.
Processors
The central processing unit (CPU) and graphics processing unit (GPU) are equally important in AI technology. GPU is designed to manipulate and alter memory to accelerate the creation of images. A cluster of GPUs is required to train a model with a training data set consisting of thousands of images. Depending on data size and performance of the model, the number of input images and SLA expected, a cluster of processors are used to meet application requirements.
Storage
To train AI and machine learning algorithms, large datasets are needed to deliver accurate decisions. This means that the demand for data storage space is significant. Managing data sets requires storage systems that can scale without limits. Your data set may not fit on the disk of a laptop, and you may need a number of SSDs and/or HDDs to store the ever-increasing training data.
AI helps make better and smarter decisions
AI technology is employed in safety-critical situations such as airports, ATM machines and aircraft operations. When decision-makers and business executives have reliable data analyses, recommendations and follow-ups through AI systems, they can make smarter decisions and better choices for their business, employees and future actions.
Automotive
In the automotive industry, several AI applications have been developed from vehicle design to marketing and sales decision-making support. For instance, AI has led to the design of smarter cars, equipped with multiple sensors that learn and identify patterns, add-on safe-drive features that warn drivers of possible collisions and lane departures. AI is used to map the decisions made by car buyers and manufacturers, and transportation services providers.
Self-driving cars
AI takes decisions by itself in a self-driving car where computer vision and machine learning plays a vital role. There are a number of interesting and challenging decision-making problems starting from autonomous driving of a single vehicle to coordination among multiple vehicles. AI algorithms take care of these problems through an autonomous system throughout the course of driving till the destination is reached.
Financial
In financial services, the model is designed to help financial service companies map buyer personas and anticipate customer behaviour. AI-enabled financial service companies can validate real-time business decisions within seconds.
Railway system
Use of AI within the rail sector is critical to making the rail experience more intuitive, and to eliminate many of the problems faced by passengers. Computational intelligence (CI) is used to forecast problems in railway maintenance, which is important and critical for safe, comfortable and economic transportation.
Before making any decision, reasoning is an essential step to explore the links between AI and decision-making. There are two aspects of decision-making: diagnosis and look-ahead. AI has many relationships with diagnosis involving expert systems, case-based reasoning, fuzzy set and rough set theories; and look-ahead reasoning involving uncertainty and preferences.
Traditionally, human operators have had to rely on inconsistent and incomplete data. With AI, data-based models and simulations are now being used to solve various problems.
Healthcare
AI is critical here because less accurate or unreliable results will put patients at risk. Therefore AI has to be accurate, and safe AI programs need to be trained, or at least constantly updated, to be able to identify new and exceptional health cases.
Remote AI-assisted robotic surgeries have been successfully carried out by many experts across the globe. With technology advancements, AI has helped personalised healthcare services, reduced the time to look for information that is critical to decision-making and facilitated better care for patients.
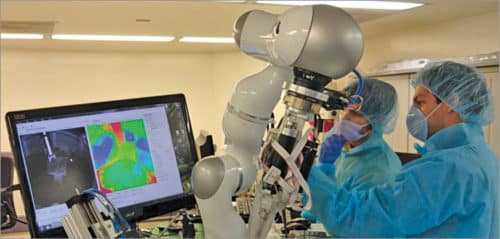
One of the more critical applications of AI in healthcare is ensuring security of data and solutions.
Conclusion
A few cases of AI in mission-critical applications including railway systems, healthcare and self-driving cars have been covered. While we are smarter and more intelligent than present machines, AI technology certainly helps us in the decision-making process to achieve better outcome. Mission-critical applications require smarter decisions, better accuracies and results. Although AI machines are used in many applications, very critical applications require the interventions of human operators.
In mission-critical AI applications, both hardware and software are equally important. Key features in mission-critical AI applications include accuracy, scale, strict SLAs and security. AI plays an important role in decision-making in various industries including railway systems, healthcare, self-driving, businesses and others.