An innovative method enables non-technical users to comprehend the reasons behind a robot’s failure and effortlessly fine-tune it to execute tasks optimally.
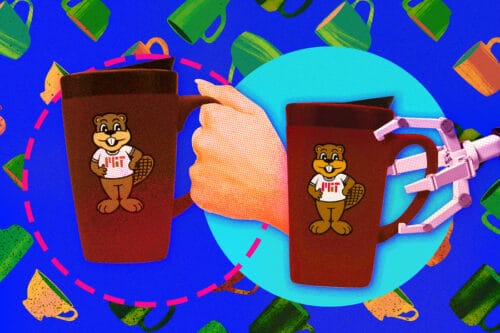
Imagine purchasing a household robot for diverse tasks. It’s factory-made and trained without knowing your home objects. Thus, when asked to fetch a mug from the kitchen table, it might not recognize your mug, leading to failure. The current robot training needs to improve its comprehension.
Researchers at MIT, New York University, and the University of California at Berkeley have developed a framework that empowers humans to efficiently instruct robots in accomplishing tasks with minimal effort. When a robot fails, an algorithm generates counterfactual explanations. The system shows these to humans, who provide feedback on the failure. Using this feedback and the explanations, the system fine-tunes the robot with new data. Fine-tuning speeds up robot learning for diverse tasks.
On-the-job training
During training, robots fail due to distribution shifts and encountering new objects and spaces. Imitation learning retrains a robot by user demonstration. However, if the user shows a white mug, the robot may wrongly assume all mugs are white. Teaching the robot to recognize mugs of any colour might require thousands of demonstrations. The framework consists of three essential stages. Initially, it introduces the task that resulted in the robot’s failure. Secondly, it gathers user demonstrations of the intended actions and generates counterfactual scenarios by exploring different features to pinpoint the required changes for the robot to achieve success. Lastly, it presents these counterfactuals to the user, collects feedback, and generates numerous augmented demonstrations, facilitating fine-tuning of the robot’s performance.
From human reasoning to robot reasoning
The researchers aimed to involve humans in training, so they evaluated their technique using human users. They applied the framework to three simulations involving robot tasks: navigation, object unlocking, and object placement. Their method facilitated faster robot learning with fewer user demonstrations. They plan to validate the framework on real robots further and reduce data creation time using generative machine learning models.
The researchers aim to have robots perform tasks similarly to humans in a semantically meaningful manner. Their goal is to enable robots to learn abstract, human-like representations effectively.