Researchers from New York have developed a novel artificial intelligence model to accurately predict the efficiency of new drug compounds on humans and speed-up drug discovery
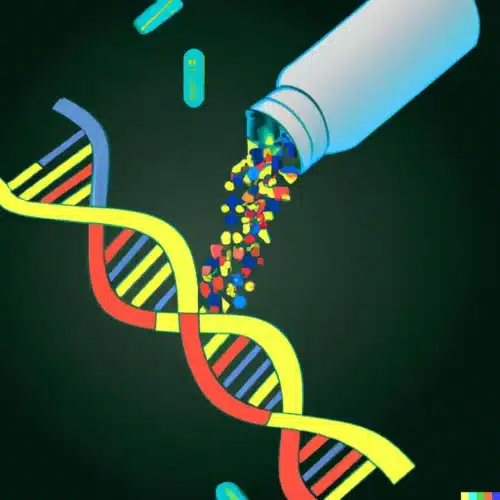
The journey of developing a new drug from a potential therapeutic compound to introducing it to the market is a very time-consuming and complex process. Since it’s unethical to do testing of new drug compounds directly on human beings, researchers use cell or tissue models as a surrogate of the human body. But, the drug effect on models does not correlate with the drug efficacy and toxicity in human patients. This results in the high costs and low productivity rates of drug discovery. Therefore, a team of researchers at the City University of New York (CUNY) Graduate Center has invented a unique artificial intelligence model that could notably enhance the accuracy of drug efficacy on human beings and decrease the time and cost of the drug development process.
“Our new machine learning model can address the translational challenge from disease models to humans,” said Lei Xie, a professor of computer science, biology, and biochemistry at the CUNY Graduate Center and Hunter College and the paper’s senior author. “CODE-AE uses biology-inspired design and takes advantage of several recent advances in machine learning. For example, one of its components uses similar techniques in Deepfake image generation.”
The study published in Nature Machine Intelligence describes the new model, called CODE-AE, that could screen novel drug compounds to accurately predict efficacy in humans. During experiments, it was able to theoretically identify personalized drugs for over 9,000 patients that could better treat their conditions. The researchers also observed that the AI model could be used to accurately predict the human side effects of drugs.
The new model can provide a workaround to the problem of having sufficient patient data to train a generalized machine learning model, said You Wu, a CUNY Graduate Center Ph.D. student and co-author of the paper. “Although many methods have been developed to utilize cell-line screens for predicting clinical responses, their performances are unreliable due to data incongruity and discrepancies,” Wu said. “CODE-AE can extract intrinsic biological signals masked by noise and confounding factors and effectively alleviated the data-discrepancy problem.”
Further, the research team aims to develop a way for CODE-AE to efficiently predict the effect of a new drug’s concentration and metabolization in human bodies.
Click here for the Published Research Paper