When we start the journey of life as new-born babies, we inherit the characteristics of our parents. We do not know what to do and when to do what. As we grow up, our parents and elders teach us how to walk, talk and take various decisions in our lives and, as time passes, we gain experience and knowledge. Finally, we start taking our own decisions based on our learning and experience.
Similarly, when we write any code to make a system do any work, the system only does what we ask it to do—it cannot think or take any extra decisions on its own, nor perform actions on that basis. Machine learning teaches the system to learn and take decisions when exposed to a new set of data on the basis of the experience it gains while performing different actions.
Machine learning is an emerging technology that is widely being implemented across all types of industries. Google’s self-driving cars, flying drones, anomaly detection and Big Data processing are among the recent examples.
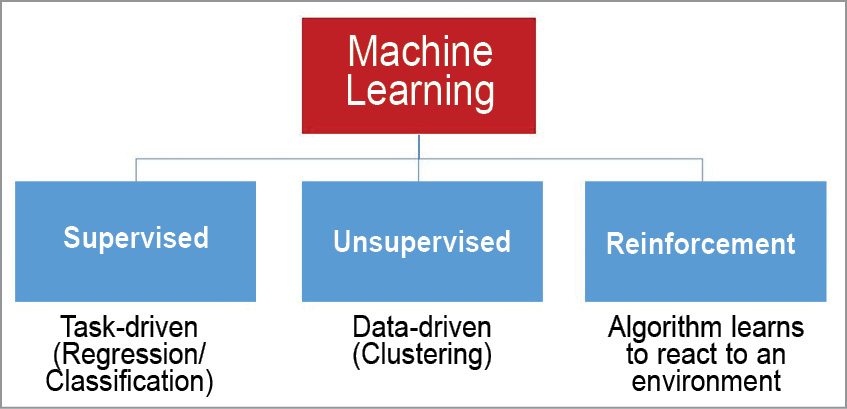
Machine learning is a type of artificial intelligence that provides computers with the ability to learn without being explicitly programmed. It uses pattern recognition and computational learning theory to study and develop algorithms (which can learn from the sets of available data), on the basis of which it takes decisions. These algorithms work by building a model (such as predictive model or neural network model) from sample inputs in order to take data-driven decisions. These models help in developing decision trees, using which the system takes its decision.
Machine learning makes use of mathematical optimisation to deliver different theories, methods and application domains for a specific field. It uses the data-mining technique to perform exploratory data analysis over a set of data in order to make predictions. This is referred to as unsupervised learning.
Machine learning helps data scientists, engineers, researchers and analysts take a reliable decision by uncovering the hidden insights acquired through the analysis of historical trends in data.
Types of machine learning
Tasks performed using machine learning are classified broadly into three categories, based on the nature of the learning signal available to a learning system (that helps take decisions).
Supervised learning.
This is a type of machine learning in which the system is presented with a set of labelled training data (inputs and their corresponding set of outputs). Now, it is the task of supervised machine learning to predict a new set of outputs for a given new set of inputs by learning or finding out a general rule or pattern that maps the given set of inputs to their corresponding outputs. The pattern or rule that helps in predicting output is generally denoted by a specific function. Supervised learning is further classified as regression and classification problems, on the basis of the methodology that is implemented to find a specific pattern.
Unsupervised learning.
This machine learning technique is implemented when there is only a set of inputs available with the system, with no corresponding outputs. Now, it is left to the system to learn and identify the pattern or rule governing the available inputs by using unsupervised learning and, further, that hypothesis or rule is used to find the output for the given set of inputs.
There can be many possible hypotheses, but the optimal one is considered for finding the output. Again, unsupervised learning technique is further classified as k means and hierarchical clustering problems, on the basis of the different techniques used to find the final hypothesis.
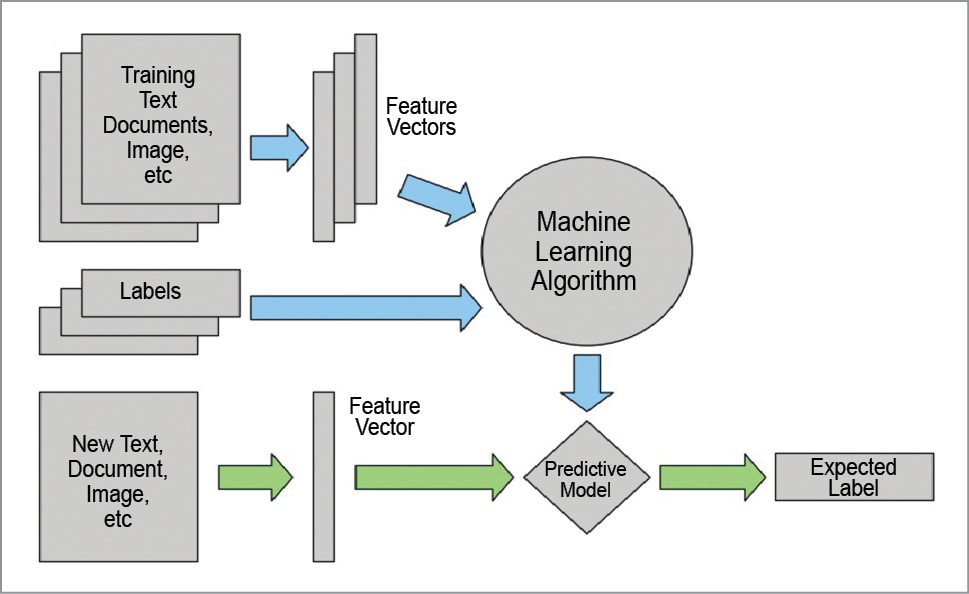
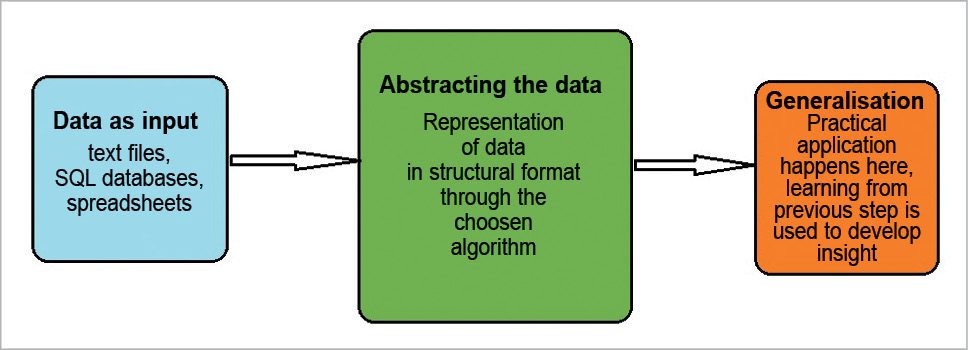
Reinforcement learning.
Here, the system is given two different sets of input data and it needs to implement reinforcement machine learning technique to learn and identify the general pattern or hypothesis in one of the given set of inputs.
There can be more than one hypothesis derived but, finally, the optimal one is used by the system to derive the output for the other set of inputs. This is like learning the rules of a game by playing against an opponent.
Implementing machine learning in real life
Let us now look at implementing machine learning in real-life scenarios. You need to check how you can teach machines to take decisions and do your work just as you would do it by applying your own sense or logic.
In the course of teaching machines, every stage of the process helps to build a better version of the machine. There are five basic steps that need to be followed prior to letting a machine perform any unsupervised task.
Collecting data.
This is one of the first and foremost steps in implementing any type of machine learning technique. Data plays a significant role in machine learning, whether it is in the form of raw data from MS Excel, Access or even text files. This step lays the foundation of future learning. We must be aware of the fact that the better the variety, volume and density of relevant data, the better will be the learning prospects for the machine.
Preparing data.
Once data is collected, check the quality of what will be fed as training data to the system. You need to spend time in order to determine the quality of data and, accordingly, take steps to fix issues such as treatment of outliers and missing data. Exploratory analysis is one such methodology used to study the differences of data in detail, thereby strengthening nutritional content.
Training a model.
This step involves selecting the appropriate algorithm and representing data in the form of a model. The final purified data is split into two parts—training and test (proportion of data depends on prerequisite requirements). The first part (training data) is used to develop the model, whereas the second part (test data) is used as reference.
Evaluating the model.
This step involves evaluation of the machine learning model you have chosen to implement. Second part of data (test data) is used to test the accuracy of the learning model. This step determines how precise the algorithm selected is, based on outcome.
There is also a better test to check accuracy of the model, which sees how the model performs on data that has not been used at all while building it.
Improving the performance.
This step may involve choosing a different model altogether or even introducing more variables to improve the efficiency of the learning model. If the model is changed, then it again needs to be evaluated and its performance checked, which is why a lot of time needs to be spent in collecting and preparing data.
Tools for implementing machine learning
In order to implement machine learning on a system for any scenario, there are enough open source tools, software or frameworks available for you to choose from, based on your preference for a specific language or environment. Let us take a look at some of these.
Shogun.
Shogun is one of the oldest and most venerable machine learning libraries available in the market. It was first developed in 1999 using C++, but now it is not limited to working in C++ only; rather, it can be used transparently in many languages and environments such as Java, C#, Python, Ruby, R, Octave, Lua and MATLAB. It is easy to use, and is quite fast at compilation and execution.
Weka.
Weka was developed at University of Waikato in New Zealand. It collects a set of Java machine learning algorithms that are engineered specifically for data mining. This GNU GPLv3-licensed collection possesses a package system, which can be used to extend its functionality. It has both official and unofficial packages available.
Weka comes with a book that explains the software and the techniques used in it. While Weka is not aimed specifically at Hadoop users, it can be used with Hadoop as well, because of the set of wrappers that have been produced for the most recent versions of it. It does not support Spark, but Clojure users can also use Weka.
CUDA-Convnet.
CUDA-Convnet is a machine learning library especially used for neural network applications. It is written in C++ in order to exploit Nvidia’s CUDA GPU processing technology. It can even be used by those who prefer Python over C++. The resulting neural nets obtained as output from this library can be saved as Python-pickled objects and, hence, can be accessed from Python.
Note that the original version of the project is no longer being developed, but has been reworked into a successor named CUDA-Convnet2. It supports multiple GPUs and Kepler-generation GPUs.
H2O.
H2O is an open source machine learning framework developed by Oxdata. H2O’s algorithms are basically geared for business processes like fraud or trend predictions. H2O can easily interact in a standalone fashion with different HDFS stores. It can be in MapReduce, on top of YARN or directly in an Amazon EC2 instance.
Hadoop Mavens can use Java for interaction with H2O, but this framework also provides bindings for R, Python and Scala. It enables cross-interaction with all libraries available on those platforms.
Applications of machine learning
The world is on the path to becoming smarter through automation of all possible manual tasks. Google and Facebook use machine learning to push their respective advertisements to relevant users. Given below are a few applications that you should know of.
Banking and financial services.
Machine learning is widely used to predict customers who are likely to be defaulters in paying credit card bills or repaying loans. This is of utmost importance as machine learning helps banks identify customers who can be given credit cards and loans.
Healthcare.
It is widely used to diagnose various deadly diseases (like cancer) on the basis of patients’ symptoms, and tallying these with the past data available for similar kinds of patients.
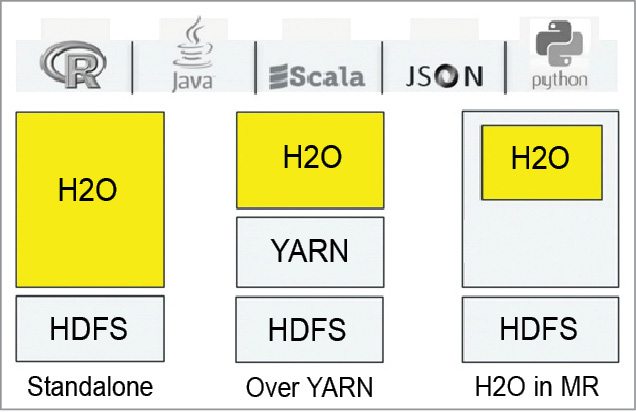
Retail.
Machine learning is used to identify products that sell fast and those that do not. It helps retailers decide on the kind of products to introduce or remove from their stock. Also, machine learning algorithms can be very effective in finding two or more products that will sell together. This is basically done to encourage customer loyalty initiatives which, in turn, help different retailers develop and maintain loyal customers. Walmart, Amazon, Big Bazaar and other such retail chains extensively make use of machine learning.
Publishing and social media.
There are publishing firms like LexisNexis and Tata McGraw Hill that make use of machine learning to run queries and fetch documents required by their users online, based on their preferences and requirements. Google and Facebook also use these techniques to rank their search outputs and news feeds. Facebook provides a list of possible friends under Friend Suggestions using this.
Robot locomotion.
Robot locomotion is a collective term used for the different methods that robots use to transport themselves from one place to other. A major challenge in this field lies in developing capabilities for different robots to autonomously decide how, when and where to move. Machine learning helps them do this quite easily.
Apart from this, there are various decisions that robots need to take instantaneously while they perform activities, which is possible using different machine learning techniques.
Game playing.
A strategy game is one in which the player’s autonomous decision-making skills are significant in determining the final outcome. Almost all strategy games require internal decision tree style of thinking, and very high situational awareness. Machine learning meets all these requirements and, hence, is widely used in gaming.
Advantages of machine learning
Machine learning techniques help the system take decisions on the basis of training data in dynamic or uncertain situations.
It can handle multi-dimensional, multi-variety data, and can extract implicit relationships within large data sets in a dynamic, complex and chaotic environment.
It allows reduction of the time cycle and improves resource utilisation. It also provides different tools for continuous quality improvement in any large or complex process.
Another advantage of machine learning techniques is the increased usability of various applications of algorithms due to source programs like Rapidminer. Machine learning allows easy application and comfortable adjustment of parameters to improve classification performance.
Challenges of machine learning
A very common challenge is acquisition of relevant data. Once available data is secured, it often has to be pre-processed depending on the requirements of the specific algorithm used, which has a critical impact on the final results.
Sometimes, interpretation of results also becomes a challenge, as these need to be interpreted according to the algorithm chosen. Different machine learning techniques can be implemented in order to let the system decide on what action it needs to take and when it needs to be taken.
Machine learning can give an edge to automation, and has already helped in making the world a lot smarter. But machines have not stopped learning, and the next level of this technology is being worked on.
Reproduced from EFY’s Open Source For You magazine
For reading more exciting tech focus articles: click here
Vivek Ratan currently works as an automation test engineer at Infosys, Pune
Nicely written article. True to its title.
Thank you Vikash, for your feedback.